Coffee Crops Analysis Using UAVs Equipped with Multispectral Cameras
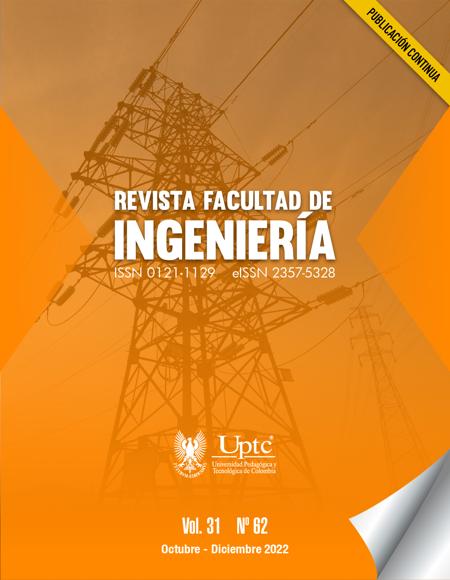
Abstract
The use of Unmanned Aerial Vehicles (UAVs) equipped with spectral cameras has increased in recent years, especially in the agricultural sector, because it allows farmers and researchers to analyze the state of a crop, i.e., health, nutrients, growth, epidemics, among other parameters. In Colombia, the coffee production sector faces several challenges, such as the need to increase the productivity, the yield, and the quality of coffee. This work estimated the health status of a Castilla variety crop located in San Joaquín, Tambo, Cauca to support the decision-making of coffee growers. For this, chlorophyll data were measured in the field with the CCM-200 plus device, multispectral images were captured with the MAPIR SURVEY 3 camera airborne on a SOLO 3DR UAV, and synthetic data were generated to increase the data set. Six vegetation indices were set, which—together with the chlorophyll values—were modeled through the implementation of simple and multiple linear regressions, decision trees, vector machines, random forests, and k-nearest neighbors. The model with the best performance and the lowest mean square error was disorder with the support vector machine. Likewise, the best performance indices in the models were CVI, GNDVI, and GCI, which are widely used in agriculture to estimate the chlorophyll of plants.
Keywords
agriculture, coffee, multispectral images, synthetic data, vegetation index, UAV
Author Biography
Natalia Arteaga-López
Rol: Conceptualization; Analysis; Writing – original draft.
Carlos Delgado-Calvache
Roles: Conceptualization; Analysis; Writing – original draft.
Juan-Fernando Casanova
Roles: Conceptualization; Methodology; Writing – edit and review.
Cristian Figeroa
Rol: Conceptualization; Methodology; Writing – edit and review.
References
- J. A. Bolaños, L. Campo, J. C. Corrales, “Characterization in the Visible and Infrared Spectrum of Agricultural Crops from a Multirotor Air Vehicle,” in Cham: Springer International Publishing, 2018, pp. 29–43. https://doi.org/10.1007/978-3-319-70187-5_3 DOI: https://doi.org/10.1007/978-3-319-70187-5_3
- J. Navia, I. Mondragon, D. Patino, J. Colorado, “Multispectral mapping in agriculture: Terrain mosaic using an autonomous quadcopter UAV,” in International Conference on Unmanned Aircraft Systems (ICUAS), pp. 1351–1358, 2016. https://doi.org/10.1109/ICUAS.2016.7502606 DOI: https://doi.org/10.1109/ICUAS.2016.7502606
- T. Duan, S. C. Chapman, Y. Guo, B. Zheng, “Dynamic monitoring of NDVI in wheat agronomy and breeding trials using an unmanned aerial vehicle,” Field Crops Research, vol. 210, no. 3, pp. 71–80, 2017. https://doi.org/10.1016/j.fcr.2017.05.025 DOI: https://doi.org/10.1016/j.fcr.2017.05.025
- S. Candiago, F. Remondino, M. de Giglio, M. Dubbini, M. Gattelli, “Evaluating Multispectral Images and Vegetation Indices for Precision Farming Applications from UAV Images,” Remote Sensing, vol. 7, no. 4, pp. 4026–4047, 2015. https://doi.org/10.3390/rs70404026 DOI: https://doi.org/10.3390/rs70404026
- E. Pino, “Los drones una herramienta para una agricultura eficiente: un futuro de alta tecnología,” Idesia (Arica), vol. 37, pp. 75–84, 2019. https://doi.org/10.4067/s0718-34292019005000402 DOI: https://doi.org/10.4067/S0718-34292019005000402
- B. Kitchenham, Procedures for Performing Systematic Reviews, 2004.
- N. Yu, L. Li, N. Schmitz, L. F. Tian, J. A. Greenberg, B. W. Diers, “Development of methods to improve soybean yield estimation and predict plant maturity with an unmanned aerial vehicle-based platform,” Remote Sensing of Environment, vol. 187, pp. 91–101, 2016. https://doi.org/10.1016/j.rse.2016.10.005 DOI: https://doi.org/10.1016/j.rse.2016.10.005
- J. Geipel, J. Link, J. Wirwahn, W. Claupein, “A Programmable Aerial Multispectral Camera System for In-Season Crop Biomass and Nitrogen Content Estimation,” Agriculture, vol. 6, no. 1, p. 4, 2016. https://doi.org/10.3390/agriculture6010004 DOI: https://doi.org/10.3390/agriculture6010004
- V. A. B. Meneses, J. M. Téllez, D. F. A. Velasquez, “Uso de drones para el análisis de imágenes multiespectrales en agricultura de precisión,” Ciencia y Tecnología Alimentaria, vol. 13, no. 1, pp. 28–40, 2015. https://doi.org/10.24054/16927125.v1.n1.2015.1647 DOI: https://doi.org/10.24054/01204211.v1.n1.2015.1647
- J. Rojas, C. Devia, E. Petro, C. Martinez, I. Mondragon, D. Patino, C. Rebolledo, J. Colorado, “Aerial mapping of rice crops using mosaicing techniques for vegetative index monitoring,” in International Conference on Unmanned Aircraft Systems (ICUAS), IEEE, pp. 846–855, 2018. https://doi.org/10.1109/ICUAS.2018.8453283 DOI: https://doi.org/10.1109/ICUAS.2018.8453283
- C. Devia, J. Rojas, E. Petro, “High-Throughput Biomass Estimation in Rice Crops Using UAV Multispectral Imagery,” Journal of Intelligent and Robotic Systems: Theory and Applications, vol. 96, no. 3–4, pp. 573–589, 2019. https://doi.org/10.1007/s10846-019-01001-5 DOI: https://doi.org/10.1007/s10846-019-01001-5
- Sngular, CRISP-DM: La metodología para poner orden en los proyectos. https://www.sngular.com/es/data-science-crisp-dm-metodologia/
- A. Azevedo, M. F. Santos, KDD, SEMMA and CRISP-DM: a parallel overview, 2008. https://recipp.ipp.pt/handle/10400.22/136
- J. Wijitdechakul, S. Sasaki, Y. Kiyoki, C. Koopipat, “UAV-based multispectral image analysis system with semantic computing for agricultural health conditions monitoring and real-time management,” in International Electronics Symposium, IEEE, pp. 459–464, 2017. https://doi.org/10.1109/ELECSYM.2016.7861050 DOI: https://doi.org/10.1109/ELECSYM.2016.7861050
- E. Aldana-Jague, G. Heckrath, A. Macdonald, B. van Wesemael, K. van Oost, “UAS-based soil carbon mapping using VIS-NIR (480–1000nm) multi-spectral imaging: Potential and limitations,” Geoderma, vol. 275, pp. 55–66, 2016. https://doi.org/10.1016/j.geoderma.2016.04.012 DOI: https://doi.org/10.1016/j.geoderma.2016.04.012
- M. O. S. Park, A. Nolan, D. Ryu, S. Fuentes, E. Hernandez, “Estimation of crop water stress in a nectarine orchard using high-resolution imagery from unmanned aerial vehicle (UAV) S,” Remote Sens (Basel), vol. 9, no. 8, p. 828, 2015. DOI: https://doi.org/10.3390/rs9080828
- I. L Castillejo-González, F. López-Granados, A. García-Ferrer, J. M. Peña-Barragán, M Jurado-Expósito, M. S. de la Orden, M. González-Audicana, “Object- and pixel-based analysis for mapping crops and their agro-environmental associated measures using QuickBird imagery,” Computers and Electronics in Agriculture, vol. 68, no. 2, pp. 207–215, 2009. https://doi.org/10.1016/j.compag.2009.06.004 DOI: https://doi.org/10.1016/j.compag.2009.06.004
- D. B. Marin, M. de Carvalho Alves, E. A. Pozza, L. L. Belan, M. L. de Oliveira Freitas, “Multispectral radiometric monitoring of bacterial blight of coffee,” Precision Agriculture, vol. 18, e0123456789, 2018. https://doi.org/10.1007/s11119-018-09623-9 DOI: https://doi.org/10.1007/s11119-018-09623-9
- D. D. W. Ren, S. Tripathi, L. K. B. Li, “Low-cost multispectral imaging for remote sensing of lettuce health,” Journal of Applied Remote Sensing, vol. 11, no. 1, e016006, 2017. https://doi.org/10.1117/1.JRS.11.016006 DOI: https://doi.org/10.1117/1.JRS.11.016006
- S. Bolanos, “Integrating GIS and remote sensing for coffee mapping Sandra,” in XII SELPER Symposium, 2006, pp. 1–11.
- T. Dong, J. Shang, J. M. Chen, J. Liu, B. Qian,B. Ma, M. Morrison, J. Malcolm, C. Zhang,Y Liu, Y. Shi, H. Pan, G. Zhou, “Assessment of Portable Chlorophyll Meters for Measuring Crop Leaf Chlorophyll Concentration,” Remote Sens (Basel), vol. 11, e2706, 2019. DOI: https://doi.org/10.3390/rs11222706
- Anonimo, Gretel. https://gretel.ai/