Using Decision Trees to Predict Critical Reading Performance.
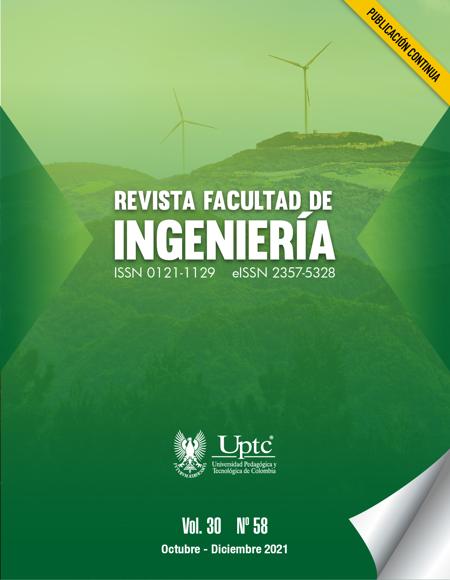
Abstract
In Colombia, all undergraduate students, regardless of the professional training program they take, must complete the general competencies sections of the Saber Pro exam that include Critical Reading, Quantitative Reasoning, Citizen Competencies, Written Communication, and English. This paper presents the application of the classification technique based on decision trees in the prediction of the performance in the Critical Reading section presented by the students of the Pontificia Universidad Javeriana Cali in the years 2017 and 2018. The CRISP methodology was used. From the socioeconomic, academic and institutional data stored in the ICFES databases, a data repository was built, cleaned and transformed. A mineable view composed of 2052 records and 17 attributes was obtained. The J48 algorithm of the Weka tool was used to build the decision tree. The score obtained in the Critical Reading section of the Saber Pro exam was taken as a class. According to the results obtained, the Philosophy, Applied Mathematics, and Medicine programs stood out for having the best performance in this test. Among the predictive variables associated with performance in the Critical Reading skill are the faculty, the age group and the student's transportation index, as three important variables related to the good or low academic performance of the students of the Universidad Javeriana Cali. The knowledge generated in this research is constituted in quality information to support the decision-making process of the university directives in order to improve the quality of the higher education offered in this institution.
Keywords
academic performance, critical reading, decision trees, J48 algorithm, Saber Pro
Author Biography
Andrea Timaran-Buchely
Roles: Data curation, Formal Analysis, Investigation, Methodology, Writing – original draft, Writing – review & editing.
Silvio-Ricardo Timarán-Pereira
Roles: Formal Analysis, Investigation, Writing – review & editing.
Arsenio Hidalgo-Troya
Roles: Formal Analysis, Investigation, Writing – review & editing.
References
- Icfes, Saber Pro: Módulos de Competencias Genéricas 2017. Instituto Colombiano para la Evaluación de la Educación Superior, Bogotá D.C., Colombia, 2017. https://www.icfes.gov.co/documents/20143/495161/Guia%20de%20orientacion%20modulos%20de%20competencias%20genericas-saber-pro-2017.pdf
- Icfes, Guía de orientación Saber Pro: Módulos de competencias genéricas, Bogotá D.C., Colombia, 2018. https://www.icfes.gov.co/documents/20143/496194/Guia%20de%20orientacion%20modulos%20de%20competencias%20genericas-saber-pro-2018.pdf
- R. Timarán, I. Hernández, J. Caicedo, A. Hidalgo, J. Alvarado, Descubrimiento de patrones de desempeño académico, Bogotá, Colombia: Ediciones Universidad Cooperativa de Colombia, 2016. DOI: https://doi.org/10.16925/9789587600490 DOI: https://doi.org/10.16925/9789587600490
- Icfes, Informe nacional de resultados Saber Pro 2012-2015, Bogotá D.C., Colombia, 2016. https://www.icfes.gov.co/documents/20143/194324/Informe%20nacional%20de%20resultados%20saber%20pro%202012%20-%202015.pdf
- L. Zapata, Factores académicos asociados al bajo rendimiento en inglés en las pruebas ECAES presentadas por los estudiantes de la Facultad de Educación en el año 2009, Grade Thesis, Fundación Universitaria Luis Amigó, Medellín, Colombia, 2011
- UNAL, Análisis de los resultados obtenidos por la Universidad Nacional de Colombia sede Bogotá en las pruebas Saber Pro 2011–2, Bogotá D.C., Colombia, 2012. https://www.unal.edu.co/diracad/evaluacion/SaberPro_2012/analisis_de_resultados.pdf
- R. Timarán, A. Calderón, J. Jiménez, Detección de Patrones de Deserción Estudiantil con Minería de Datos, San Juan de Pasto, Colombia: Editorial Universidad de Nariño, 2017
- S. Valero, A. Vargas, M. Alonso, Minería de datos: predicción de la deserción escolar mediante el algoritmo de árboles de decisión y el algoritmo de los k vecinos más cercanos, 2005. http://fcaenlinea.unam.mx/anexos/1566/1566_u6_act1b.pdf.
- H. Escobar, M. Alcívar, C. Márquez, C. Escobar, “Implementación de Minería de Datos en la Gestión Académica de las Instituciones de Educación Superior,” Didasc@lia: Didáctica y Educación, vol. 8, no. 3, pp. 203-212, 2017
- A. Azevedo, M. Santos, “KDD, SEMMA and CRISP-DM: a parallel overview,” in Proceedings of IADIS European Conference on Data Mining, pp. 182-185, 2008
- J. Villena, CRISP-DM: La metodología para poner orden en los proyectos de Data Science, 2016. https://data.sngular.team/es/art/25/crisp-dm-la-metodologia-para-poner-orden-en-los-proyectos-de-data-science
- J. Hernández, M. Ramírez, C. Ferri, Introducción a la Minería de Datos. Madrid, España: Editorial Pearson Educación S.A., 2005
- J. Han, M. Kamber, Data Mining: Concepts and Techniques. San Francisco, USA: Morgan Kaufmann Publishers, 2001
- K. Sattler, O. Dunemann, “SQL Database Primitives for Decision Tree Classifiers,” in 10th ACM International Conference on Information and Knowledge Management, pp. 379-386, 2001 DOI: https://doi.org/10.1145/502585.502650
- R. Timarán, J. Caicedo, A. Hidalgo, Aplicación de la minería de datos en la detección de patrones de desempeño académico en las pruebas Saber Pro, San Juan de Pasto, Colombia: Editorial Universidad de Nariño, 2021
- E. Hernández, R. Lorente, Minería de datos aplicada a la detección de Cáncer de Mama. Universidad Carlos III, Madrid, Spain, 2009. http://www.it.uc3m.es/jvillena/irc/practicas/08-09/14.pdf
- I. Witten, E. Frank, M. Hall, Data Mining: Practical Machine Learning Tools and Techniques (Third Edition). New York, USA: Morgan Kaufmann, 2011. DOI: https://doi.org/10.1016/C2009-0-19715-5 DOI: https://doi.org/10.1016/C2009-0-19715-5
- J. R. Quinlan, Programs for Machine Learning. San Francisco, USA: Morgan Kaufmann Publishers, 1993
- M. García, A. Álvarez, Análisis de Datos en WEKA: Pruebas de Selectividad, 2010. http://www.it.uc3m.es/jvillena/irc/practicas/06-07/28.pdf