Comparison of Kernel Functions in the Classification of Irradiance Zones from Multispectral Satellite Images
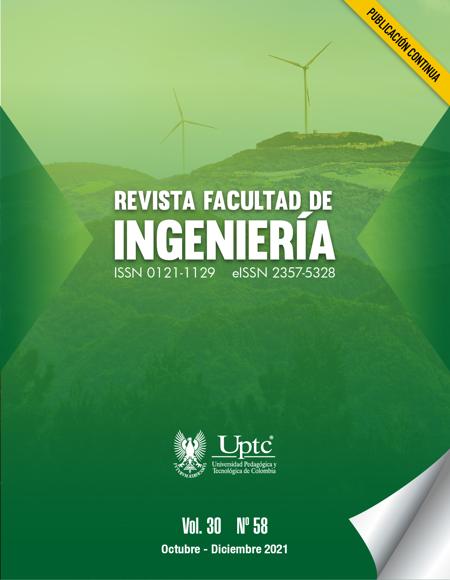
Abstract
Due to the growing energy demand and the eminent global warming, there is special interest in the prediction of irradiance based on the reflectance obtained from satellites such as NASA Landsat, since it allows to know where it is more efficient to place photovoltaic receivers. Although there are studies for obtaining regression models with alternative Kernel functions, their performance for classification models is unknown and it is here where this research focuses. The study couples alternative Kernel functions to the support vector machines (SVM) algorithm for classification problems, where the best configuration for these algorithms is explored to finally obtain a set of irradiance maps zoned by class.
Keywords
Kernel functions, multispectral satellite images, Landsat, Support Vector Machines, Classification, photovoltaic energy
Author Biography
Dalila-Mercedes Pachajoa
Roles: Formal Analysis, Data Preprocessing, Research, Methodology, Software, Validation, Visualization, Writing-Original Draft, Writing-Revision and Editing.
Héctor Mora-Paz
Roles: Formal Analysis, Data Preprocessing, Research, Methodology, Software, Validation, Visualization, Writing-Original Draft, Writing-Revision and Editing.
Dagoberto Mayorca-Torres
Roles: Conceptualization, Methodology, Validation, Writing-Original Draft, Writing-Revision and Editing.
References
- M. Zorzi, A. Chiuso, "The harmonic analysis of kernel functions" Automatic, vol. 94, pp. 125-137, 2018. https://doi.org/10.1016/j.automatica.2018.04.015 DOI: https://doi.org/10.1016/j.automatica.2018.04.015
- L. A. Belanche Muñoz, "Developments in kernel design", UPCommons, pp. 369-378, 2013. https://upcommons.upc.edu/handle/2117/23278
- C. Spiegeler, J. I. Cifuentes, Definition and information on renewable energies, Grade Thesis, Universidad de San Carlos de Guatemala, Guatemala, 2016
- J. Gómez Ramírez, Photovoltaic solar energy in Colombia: potentials, antecedents and perspectives, Grade Thesis, Universidad Santo Tomás, Bogotá D.C., Colombia, 2018. https://repository.usta.edu.co/handle/11634/10312
- Unidad de Planeación Minero Energética, Integración de las energías renovables no convencionales en Colombia, Bogotá D.C., Colombia, 2015
- O. E. Cabrera Rosero, A. D. Pantoja Bucheli, " Analysis of the wind resource using R in non-interconnected zones (ZNI) of the department of Nariño (Colombia)," in Latin American Conference on the Use of R in Research + Development, 2018. http://sedici.unlp.edu.ar/handle/10915/72585
- H. A. Mora-Paz, Comparison of kernels on prediction of supply of alternative energy sources, Master Thesis, Universidad Internacional de la Rioja, Logroño, Spain, 2021. https://reunir.unir.net/handle/123456789/10020
- O. Cabrera, B. Champutiz, A. Calderon, A. Pantoja, "Landsat and MODIS satellite image processing for solar irradiance estimation in the department of Narino-Colombia," in XXI Symposium on Signal Processing, Images and Artificial Vision (STSIVA), 2016, pp. 1-6. https://doi.org/10.1109/STSIVA.2016.7743306 DOI: https://doi.org/10.1109/STSIVA.2016.7743306
- S. H. Monger, E. R. Morgan, A. R. Dyreson, T. L. Acker, "Applying the kriging method to predicting irradiance variability at a potential PV power plant", Renewable Energy, vol. 86, pp. 602-610, 2016. https://doi.org/10.1016/j.renene.2015.08.058 DOI: https://doi.org/10.1016/j.renene.2015.08.058