Sistema Inteligente para el manejo de Malezas en el cultivo de Piña con Conceptos de Agricultura de Precisión
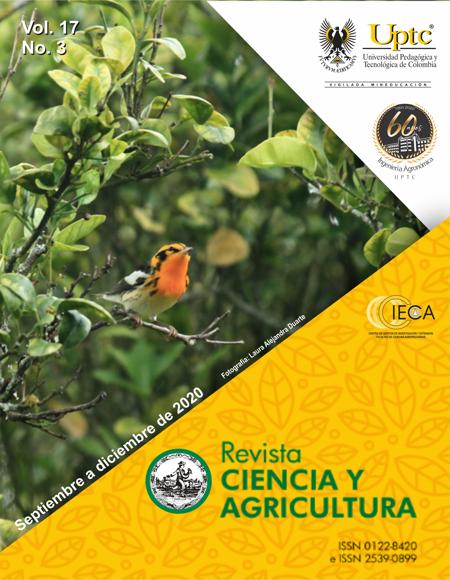
Resumen
La agricultura de precisión busca la aplicación de insumos en cultivos agrícolas en el lugar, el momento y la cantidad adecuados. El manejo de malezas específico del sitio es una estrategia de agricultura de precisión que permite la reducción en la aplicación de herbicidas, minimizando costos de insumos, con efectos positivos para el medioambiente. El objetivo de este artículo es mostrar los avances en el desarrollo de un sistema inteligente para la detección de malezas y aplicación de herbicida en un cultivo de piña con conceptos de agricultura de precisión. El prototipo utiliza un sistema de visión artificial para la adquisición de la reflectancia en las plantas en el espectro del visible y un sistema embebido que permite el procesamiento de las imágenes en tiempo real como mecanismo de detección de maleza. El prototipo cuenta con un sistema de fumigación automático, el cual emula la aplicación del herbicida selectivo; lo que en conjunto es implementado sobre un vehículo terrestre que realiza su recorrido entre los surcos de un cultivo de piña. El algoritmo de detección de malezas para el cultivo de piña tuvo una eficiencia de más del 80 %, obteniendo así resultados satisfactorios y el cumplimiento de requerimiento para la detección y aplicación de insumo solo en los lugares en donde se necesita.
Palabras clave
Agricultura de Precisión, cultivo agrícola, malezas, sistema de detección, piña
Biografía del autor/a
ANDRÉS FERNANDO JIMÉNEZ LÓPEZ
Ingeniero electrónico de la Universidad Pedagógica y Tecnológica de Colombia. Magister en Ciencias Básicas - Física de la Universidad Nacional de Colombia. Actualmente soy docente de planta de la Universidad de los Llanos, del Departamento de Matemáticas y Física de la Facultad de Ciencias Básicas e Ingeniería.
Referencias
Abouzahir, S., Sadik, M., y Sabir, E. (2017). Iot-empowered smart agriculture: A real-time light-weight embedded segmentation system. In International Symposium on Ubiquitous Networking, Springer, Cham, May, 2017, pp. 319-332. https://doi.org/10.1007/978-3-319-68179-5_28
Abouzahir, S., Sadik, M., y Sabir, E. (2018). Enhanced Approach for Weeds Species Detection Using Machine Vision. In 2018 International Conference on Electronics, Control, Optimization and Computer Science (ICECOCS) - IEEE, Kenitra, Morocco, 1-6. Diciembre 2018. 1-6. https://doi.org/10.1109/ICECOCS.2018.8610505
Agrawal, K. N., Singh, K., Bora, G. C., y Lin, D. (2012). Weed recognition using image-processing technique based on leaf parameters, J. Agric. Sci. Technol. B, 2(8B), 899. ISSN: 2161-6264. http://www.davidpublishing.org/show.h...
Bakhshipour, A., y Jafari, A. (2018). Evaluation of support vector machine and artificial neural networks in weed detection using shape features. Computers and Electronics in Agriculture, 145, 153-160. ISSN: 0168-1699. https://doi.org/10.1016/j.compag.2017.12.032
Barrero, O., Rojas, D., Gonzalez, C., y Perdomo, S. (2016). Weed detection in rice fields using aerial images and neural networks, In 2016 XXI Symposium on Signal Processing, Images and Artificial Vision (STSIVA)- IEEE, Bucaramanga, Colombia, August, 2016, 1-4. https://doi.org/10.1109/STSIVA.2016.7743317
Bradski, G., y Kaehler, A. (2008). Learning OpenCV: Computer vision with the OpenCV library. " O'Reilly Media, Inc.". ISBN: 978-0-596-51613-0
Brenes-Prendas, S., y Agüero-Alvarado, R. (2007). Reconocimiento taxonómico de arvenses y descripción de su manejo, en cuatro fincas productoras de piña (Ananas comosus l.) en Costa Rica. Agronomía mesoamericana, 18(2), 239-246. ISSN: 1021-7444. http://www.redalyc.org/articulo.oa?id=43718209
Di Cicco, M., Potena, C., Grisetti, G., y Pretto, A. (2017). Automatic model based dataset generation for fast and accurate crop and weeds detection, In 2017 IEEE/RSJ International Conference on Intelligent Robots and Systems (IROS) – IEEE, Vancouver, BC, Canada, 5188-5195, September 2017, 5188-5195. https://doi.org/10.1109/IROS.2017.8206408
Gee, C., Bossu, J., Jones, G., y Truchetet, F. (2008). Detecting crops and weeds in precision agriculture, SPIE Newsroom, 2008, https://doi.org/10.1117/2.1200809.1226
Lottes, P., Behley, J., Chebrolu, N., Milioto, A., y Stachniss, C. (2018). Joint stem detection and crop-weed classification for plant-specific treatment in precision farming, In 2018 IEEE/RSJ International Conference on Intelligent Robots and Systems (IROS) – IEEE, Madrid, Spain, October, 2018, 8233-8238. https://doi.org/10.1109/IROS.2018.8593678
Lottes, P., Hoeferlin, M., Sander, S., Müter, M., Schulze, P., y Stachniss, L. C. (2016). An effective classification system for separating sugar beets and weeds for precision farming applications. In 2016 IEEE International Conference on Robotics and Automation (ICRA) – IEEE, Stockholm, Sweden, May, 2016, 5157-5163. https://doi.org/10.1109/ICRA.2016.7487720
Pérez-Ortiz, M., Gutiérrez, P. A., Peña, J. M., Torres-Sánchez, J., López-Granados, F., y Hervás-Martínez, C. (2016). Machine learning paradigms for weed mapping via unmanned aerial vehicles. In 2016 IEEE symposium series on computational intelligence (SSCI) – IEEE, Athens, Greece, December, 2016, pp. 1-8. https://doi.org/10.1109/SSCI.2016.7849987
Potena, C., Nardi, D., y Pretto, A. (2016). Fast and accurate crop and weed identification with summarized train sets for precision agriculture. In International Conference on Intelligent Autonomous Systems, Springer, Cham, July, 2016, pp. 105-121. https://doi.org/10.1007/978-3-319-48036-7_9
Rehman, T. U., Zaman, Q. U., Chang, Y. K., Schumann, A. W., y Corscadden, K. W. (2019). Development and field evaluation of a machine vision based in-season weed detection system for wild blueberry, Comput. Electron. Agric., 162, 1-13. ISSN: 0168-1699. https://doi.org/10.1016/j.compag.2019.03.023
Russell, S. J., y Norvig, P. (2016). Artificial intelligence: a modern approach. Malaysia.
Sa, I., Chen, Z., Popović, M., Khanna, R., Liebisch, F., Nieto, J., y Siegwart, R. (2017). weednet: Dense semantic weed classification using multispectral images and mav for smart farming, IEEE Robotics and Automation Letters, 3(1), 588-595. ISSN: 2377-3766. https://doi.org/10.1109/LRA.2017.2774979
Sandino, J., y Gonzalez, F. (2018). A novel approach for invasive weeds and vegetation surveys using uas and artificial intelligence, In 2018 23rd International Conference on Methods y Models in Automation y Robotics (MMAR)-IEEE, Miedzyzdroje, Poland, August, 2018, 515-520. https://doi.org/10.1109/MMAR.2018.8485874
Segura, M. A. M. (2015). Uso de agroquímicos en la producción intensiva de piña en Costa Rica. Pensamiento actual, 15(25), 183-195. ISSN electrónico: 2215-3586. https://revistas.ucr.ac.cr/index.php/pensamiento-actual/article/view/22604/24028
Siddiqi, M. H., Ahmad, I., y Sulaiman, S. B. (2009). Edge link detector based weed classifier, In 2009 International Conference on Digital Image Processing - IEEE, Bangkok, Thailand, March, 2009, 255-259. https://doi.org/10.1109/ICDIP.2009.64
Tang, J. L., Chen, X. Q., Miao, R. H., y Wang, D. (2016). Weed detection using image processing under different illumination for site-specific areas spraying. Computers and Electronics in Agriculture, 122, 103-111. ISSN: 0168-1699. https://doi.org/10.1016/j.compag.2015.12.016
Utstumo, T., Urdal, F., Brevik, A., Dørum, J., Netland, J., Overskeid, Ø., ... y Gravdahl, J. T. (2018). Robotic in-row weed control in vegetables. Computers and electronics in agriculture, 154, 36-45. ISSN: 01681699. https://doi.org/10.1016/j.compag.2018.08.043
Wagstaff, K. L., y Liu, G. Z. (2018). Automated Classification to Improve the Efficiency of Weeding Library Collections. The Journal of Academic Librarianship, 44(2), 238-247. ISSN: 0099-1333. https://doi.org/10.1016/j.acalib.2018.02.001
Weatherspark.com (2019). [online] Disponible en: https://weatherspark.com/y/24273/Average-Weather-in-Villavicencio-Colombia-Year-Round [Acceso 01 febrero 2019]. https://es.weatherspark.com.
Yang, C. C., Prasher, S. O., Landry, J. A., y Ramaswamy, H. S. (2003). Development of a herbicide application map using artificial neural networks and fuzzy logic. Agricultural systems, 76(2), 561-574. ISSN: 0308-521X. https://doi.org/10.1016/S0308-521X(01)00106-8
Zhang, W., y Wei, X. (2019). A review on weed detection using ground-based machine vision and image processing techniques. Computers and electronics in agriculture, 158, 226-240. ISSN: 0168-1699. https://doi.org/10.1016/j.compag.2019.02.005
Zheng, Y., Zhu, Q., Huang, M., Guo, Y., y Qin, J. (2017). Maize and weed classification using color indices with support vector data description in outdoor fields. Computers and Electronics in Agriculture, 141, 215-222. ISSN: 0168-1699. https://doi.org/10.1016/j.compag.2017.07.028Abouzahir, S., Sadik, M. & Sabir, E. (2017). Iot-empowered Smart Agriculture: A Real-Time Light-Weight Embedded Segmentation System. In International Symposium on Ubiquitous Networking, Springer, Cham, May 2017, pp. 319-332. https://doi.org/10.1007/978-3-319-68179-5_28
Abouzahir, S., Sadik, M. & Sabir, E. (2018). Enhanced Approach for Weeds Species Detection Using Machine Vision. In 2018 International Conference on Electronics, Control, Optimization and Computer Science (ICECOCS)- IEEE, Kenitra, Morocco, 1-6. December 2018. 1-6. https://doi.org/10.1109/ICECOCS.2018.8610505
Agrawal, K. N., Singh, K., Bora, G. C. & Lin, D. (2012). Weed Recognition Using Image-Processing Technique Based on Leaf Parameters. J. Agric. Sci. Technol. B, 2(8B), 899. http://www.davidpublishing.org/show.h...
Bakhshipour, A. & Jafari, A. (2018). Evaluation of Support Vector Machine and Artificial Neural Networks in Weed Detection Using Shape Features. Computers and Electronics in Agriculture, 145, 153-160. https://doi.org/10.1016/j.compag.2017.12.032
Barrero, O., Rojas, D., González, C. & Perdomo, S. (2016). Weed Detection in Rice Fields Using Aerial Images and Neural Networks. In 2016 XXI Symposium on Signal Processing, Images and Artificial Vision (STSIVA)- IEEE, Bucaramanga, Colombia, August 2016, 1-4. https://doi.org/10.1109/STSIVA.2016.7743317
Bastidas, D. A., Guerrero, J. A. & Wyckhuys, K. (2013). Residuos de plaguicidas en cultivos de pasifloras en regiones de alta producción en Colombia. Revista Colombiana de Química, 4(22), 39-47.
Bradski, G. & Kaehler, A. (2008). Learning OpenCV: Computer Vision with the OpenCV Library. O'Reilly Media, Inc.
Brenes-Prendas, S. & Agüero-Alvarado, R. (2007). Reconocimiento taxonómico de malezas y descripción de su manejo, en cuatro fincas productoras de piña (Ananas comosus l.) en Costa Rica. Agronomía Mesoamericana, 18(2), 239-246. http://www.redalyc.org/articulo.oa?id=43718209
Di Cicco, M., Potena, C., Grisetti, G. & Pretto, A. (2017). Automatic Model Based Dataset Generation for Fast and Accurate Crop and Weeds Detection. In 2017 IEEE/RSJ International Conference on Intelligent Robots and Systems (IROS)–IEEE, Vancouver, BC, Canada, 5188-5195, September 2017, 5188-5195. https://doi.org/10.1109/IROS.2017.8206408
Farooq, A., Hu, J. & Jia, X. (2018). Weed Classification in Hyperspectral Remote Sensing Images Via Deep Convolutional Neural Network. In IGARSS 2018-2018 IEEE International Geoscience and Remote Sensing Symposium – IEEE, Valencia, Spain, July, 2018, pp. 3816-3819. https://doi.org/10.1109/IGARSS.2018.8518541
Gee, C., Bossu, J., Jones, G. & Truchetet, F. (2008). Detecting Crops and Weeds in Precision Agriculture, SPIE Newsroom, 2008, https://doi.org/10.1117/2.1200809.1226
Jaramillo-Colorado, B. E., Palacio-Herrera, F. & Pérez-Sierra, I. (2016). Residuos de pesticidas organofosforados en frutas obtenidas de plazas de mercado y supermercados en Cartagena, Colombia. Revista Ciencias Técnicas Agropecuarias, 25(4), 39-46.
Lottes, P., Behley, J., Chebrolu, N., Milioto, A. & Stachniss, C. (2018). Joint Stem Detection and Crop-Weed Classification for Plant-Specific Treatment in Precision Farming. In 2018 IEEE/RSJ International Conference on Intelligent Robots and Systems (IROS)–IEEE, Madrid, Spain, October 2018, 8233-8238. https://doi.org/10.1109/IROS.2018.8593678
Lottes, P., Hoeferlin, M., Sander, S., Müter, M., Schulze, P., & Stachniss, L. C. (2016). An Effective Classification System for Separating Sugar Beets and Weeds for Precision Farming Applications. In 2016 IEEE International Conference on Robotics and Automation (ICRA)–IEEE, Stockholm, Sweden, May, 2016, 5157-5163. https://doi.org/10.1109/ICRA.2016.7487720
Pérez-Ortiz, M., Gutiérrez, P. A., Peña, J. M., Torres-Sánchez, J., López-Granados, F. & Hervás-Martínez, C. (2016). Machine Learning Paradigms for Weed Mapping Via Unmanned Aerial Vehicles. In 2016 IEEE Symposium Series on computational intelligence (SSCI)–IEEE, Athens, Greece, December, 2016, pp. 1-8. https://doi.org/10.1109/SSCI.2016.7849987
Potena, C., Nardi, D. & Pretto, A. (2016). Fast and Accurate Crop and Weed Identification with Summarized Train Sets for Precision Agriculture. In International Conference on Intelligent Autonomous Systems, Springer, Cham, July, 2016, pp. 105-121. https://doi.org/10.1007/978-3-319-48036-7_9
Rehman, T. U., Zaman, Q. U., Chang, Y. K., Schumann, A. W. & Corscadden, K. W. (2019). Development and Field Evaluation of a Machine Vision Based In-Season Weed Detection System for Wild Blueberry. Comput. Electron. Agric., 162, 1-13. https://doi.org/10.1016/j.compag.2019.03.023
Reyes, Y., Vergara, I., Torres, O., Lagos, M. D. & Jiménez, E. E. G. (2016). Contaminación por metales pesados: Implicaciones en salud, ambiente y seguridad alimentaria. Ingeniería Investigación y Desarrollo: I2+ D, 16(2), 66-77.
Russell, S. J. & Norvig, P. (2016). Artificial Intelligence: A Modern Approach (3th ed.). Pearson.
Sa, I., Chen, Z., Popović, M., Khanna, R., Liebisch, F., Nieto, J. & Siegwart, R. (2017). Weednet: Dense Semantic Weed Classification Using Multispectral Images and Mav for Smart Farming. IEEE Robotics and Automation Letters, 3(1), 588-595. https://doi.org/10.1109/LRA.2017.2774979
Sandino, J. & González, F. (2018). A Novel Approach for Invasive Weeds and Vegetation Surveys Using UAS and Artificial Intelligence. In 2018 23rd International Conference on Methods y Models in Automation y Robotics (MMAR)-IEEE, Miedzyzdroje, Poland, August 2018, 515-520. https://doi.org/10.1109/MMAR.2018.8485874
Segura, M. A. M. (2015). Uso de agroquímicos en la producción intensiva de piña en Costa Rica. Pensamiento Actual, 15(25), 183-195. https://revistas.ucr.ac.cr/index.php/pensamientoactual/article/view/22604/24028
Siddiqi, M. H., Ahmad, I. & Sulaiman, S. B. (2009). Edge Link Detector Based Weed Classifier. In 2009 International Conference on Digital Image Processing-IEEE, Bangkok, Thailand, March 2009, 255-259. https://doi.org/10.1109/ICDIP.2009.64
Tang, J. L., Chen, X. Q., Miao, R. H. & Wang, D. (2016). Weed Detection Using Image Processing under Different Illumination for Site-Specific Areas Spraying. Computers and Electronics in Agriculture, 122, 103-111. https://doi.org/10.1016/j.compag.2015.12.016
Utstumo, T., Urdal, F., Brevik, A., Dørum, J., Netland, J., Overskeid, Ø. & Gravdahl, J. T. (2018). Robotic In-Row Weed Control in Vegetables. Computers and Electronics in Agriculture, 154, 36-45. https://doi.org/10.1016/j.compag.2018.08.043
Wang, A., Zhang, W. & Wei, X. (2019). A review on weed detection using ground-based machine vision and image processing techniques. Computers and Electronics in Agriculture, 158, 226-240. https://doi.org/10.1016/j.compag.2019.02.005
Wagstaff, K. L. & Liu, G. Z. (2018). Automated Classification to Improve the Efficiency of Weeding Library Collections. The Journal of Academic Librarianship, 44(2), 238-247. https://doi.org/10.1016/j.acalib.2018.02.001
Weatherspark.com (2019). [online] https://weatherspark.com/y/24273/Average-Weather-in-Villavicencio-Colombia-Year-Round
Yang, C. C., Prasher, S. O., Landry, J. A. & Ramaswamy, H. S. (2003). Development of a Herbicide Application Map Using Artificial Neural Networks and Fuzzy Logic. Agricultural Systems, 76(2), 561-574. https://doi.org/10.1016/S0308-521X(01)00106-8
Zhang, W. & Wei, X. (2019). A Review on Weed Detection Using Ground-Based Machine Vision and Image Processing Techniques. Computers and Electronics in Agriculture, 158, 226-240. https://doi.org/10.1016/j.compag.2019.02.005
Zheng, Y., Zhu, Q., Huang, M., Guo, Y. & Qin, J. (2017). Maize and Weed Classification Using Color Indices with Support Vector Data Description in Outdoor Fields. Computers and Electronics in Agriculture, 141, 215-222. https://doi.org/10.1016/j.compag.2017.07.028