Encontrar temas en escritura creativa sobre la preservación ambiental para mejorar las estrategias de enseñanza: un caso de estudio en una escuela de primaria de Colombia
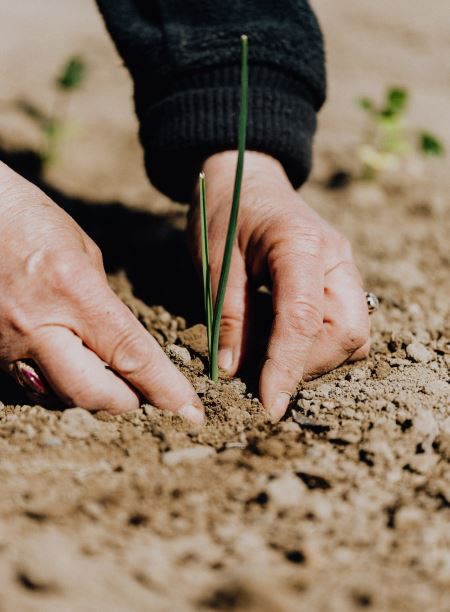
Abstract
In this research, essays on trees preservation of fourth grade students (elementary school from Colombia) were evaluated with Latent Dirichlet Allocation (LDA). The objective was extracting the fundamental topics, to understand the students’ behavior and awareness towards the environment from the creative writing. The computational results suggest the student’s reflections on environment preservation are focused on five main topics in: Teach-Learn to care for the environment, Explore-discover the environment, Well-being of the environment, Concern for the environment, and Restoration and conservation of the environment. This text analysis by LDA can complement the manual analysis of teachers, avoiding the veracity bias and allowing the enhancement of teaching strategies.
Keywords
reflective writing, topic modeling, LDA model, primary school students, technology application
References
- Aletras, N., & Stevenson, M. (2013). Evaluating topic coherence using distributional semantics. In Proceedings of the 10th International Conference on Computational Semantics, IWCS 2013 - Long Papers.
- Anandarajan, M., Hill, C., & Nolan, T. (2019). Cluster Analysis: Modeling Groups in Text. https://doi.org/10.1007/978-3-319-95663-3_7
- Balyan, R., McCarthy, K. S., & McNamara, D. S. (2020). Applying Natural Language Processing and Hierarchical Machine Learning Approaches to Text Difficulty Classification. International Journal of Artificial Intelligence in Education, 30(3), 337–370. https://doi.org/10.1007/s40593-020-00201-7
- Bhardwaj, A., Reddy, M., Setlur, S., Govindaraju, V., & Ramachandrula, S. (2010). Latent Dirichlet Allocation based Writer Identification in Offline handwriting. ACM International Conference Proceeding Series, 357–362. https://doi.org/10.1145/1815330.1815376
- Blei, D. M., Ng, A. Y., & Edu, J. B. (2003). Latent Dirichlet Allocation Michael I. Jordan. In Journal of Machine Learning Research (Vol. 3).
- Blei, D. M., Ng, A. Y., & Jordan, M. T. (2002). Latent dirichlet allocation. In Advances in Neural Information Processing Systems.
- Chang, J., Boyd-Graber, J., Gerrish, S., Wang, C., & Blei, D. M. (2009). Reading tea leaves: How humans interpret topic models. In Advances in Neural Information Processing Systems 22 - Proceedings of the 2009 Conference. http://rexa.info
- Chen, B., Chen, X., & Xing, W. (2015). “Twitter Archeology” of Learning Analytics and Knowledge Conferences. https://doi.org/10.1145/2723576.2723584
- Chen, Y., Yu, B., Zhang, X., & Yu, Y. (2016). Topic modeling for evaluating students’ reflective writing: A case study of pre-Service teachers’ journals. ACM International Conference Proceeding Series, 25-29-Apri, 1–5. https://doi.org/10.1145/2883851.2883951
- Chuang, J., Gupta, S., Manning, C. D., & Heer, J. (2013). Topic model diagnostics: Assessing domain relevance via topical alignment. In 30th International Conference on Machine Learning, ICML 2013 (Issue PART 2). http://vis.stanford.edu/topic-diagnostics
- Erkens, M., Bodemer, D., & Hoppe, H. U. (2016). Improving collaborative learning in the classroom: Text mining based grouping and representing. International Journal of Computer-Supported Collaborative Learning, 11(4), 387–415. https://doi.org/10.1007/s11412-016-9243-5
- Evaluation of Topic Modeling: Topic Coherence | DataScience+. (n.d.). Retrieved January 19, 2021, from https://datascienceplus.com/evaluation-of-topic-modeling-topiccoherence/
- Ezen-Can, A., & Boyer, K. E. (n.d.). Unsupervised Classification of Student Dialogue Acts With Query-Likelihood Clustering.
- Faustmann, G. (2018). Improved learning of academic writing reducing complexity by modeling academic texts. CSEDU 2018 - Proceedings of the 10th International Conference on Computer Supported Education, 1, 447–453. https://doi.org/10.5220/0006792204470453
- Fischer, C., Pardos, Z. A., Baker, R. S., Williams, J. J., Smyth, P., Yu, R., Slater, S., Baker, R., & Warschauer, M. (2020). Mining Big Data in Education: Affordances and Challenges. Review of Research in Education, 44(1), 130–160. https://doi.org/10.3102/0091732X20903304
- Greene, D., O’Callaghan, D., & Cunningham, P. (2014). How many topics? Stability analysis for topic models. Lecture Notes in Computer Science (Including Subseries Lecture Notes in Artificial Intelligence and Lecture Notes in Bioinformatics), 8724 LNAI(PART 1), 498–513. https://doi.org/10.1007/978-3-662-44848-9_32
- Gui, L., Leng, J., Pergola, G., Zhou, Y., Xu, R., & He, Y. (n.d.). Neural Topic Model with Reinforcement Learning. Retrieved January 25, 2021, from http://mallet.cs.umass.edu/import-stoplist.php
- Jelodar, H., Wang, Y., Yuan, C., Feng, X., Jiang, X., Li, Y., & Zhao, L. (2019). Latent Dirichlet allocation (LDA) and topic modeling: models, applications, a survey. Multimedia Tools and Applications, 78(11), 15169–15211. https://doi.org/10.1007/s11042-018-6894-4
- Kakkonen, T., Myller, N., & Sutinen, E. (2006). Applying latent Dirichlet allocation to automatic essay grading. Lecture Notes in Computer Science (Including Subseries Lecture Notes in Artificial Intelligence and Lecture Notes in Bioinformatics), 4139 LNAI, 110–120. https://doi.org/10.1007/11816508_13
- Kherwa, P., & Bansal, P. (2018). Latent Semantic Analysis: An Approach to Understand Semantic of Text. International Conference on Current Trends in Computer, Electrical, Electronics and Communication, CTCEEC 2017, 870–874. https://doi.org/10.1109/CTCEEC.2017.8455018
- Landauer, T. K., McNamara, D., Dennis, S., & Kintsch, W. (2011). The Handbook of Latent Semantic Analysis.
- Lei, L., Deng, Y., & Liu, D. (2020). Examining research topics with a dependency-based noun phrase extraction method: a case in accounting. Library Hi Tech. https://doi.org/10.1108/LHT-12-2019-0247
- Lenhart, J., Lenhard, W., Vaahtoranta, E., & Suggate, S. (2020). More than words: Narrator engagement during storytelling increases children’s word learning, story comprehension, and on-task behavior. Early Childhood Research Quarterly, 51, 338–351. https://doi.org/10.1016/j.ecresq.2019.12.009
- Letsche, T. A., & Berry, M. W. (1997). Large-scale information retrieval with latent semantic indexing. Information Sciences, 100(1–4), 105–137. https://doi.org/10.1016/S0020-0255(97)00044-3
- Liu, S., Peng, X., Cheng, H. N. H., Liu, Z., Sun, J., & Yang, C. (2019). Unfolding Sentimental and Behavioral Tendencies of Learners’ Concerned Topics From Course Reviews in a MOOC. Journal of Educational Computing Research, 57(3), 670–696. https://doi.org/10.1177/0735633118757181
- Liu, Z. (2013). High Performance Latent Dirichlet Allocation for Text Mining.
- Louvigne, S., Kato, Y., Rubens, N., & Ueno, M. (2014). Goal-based messages recommendation utilizing latent dirichlet allocation. Proceedings - IEEE 14th International Conference on Advanced Learning Technologies, ICALT 2014, 464–468. https://doi.org/10.1109/ICALT.2014.138
- Mimno, D., Wallach, H. M., Talley, E., Leenders, M., & Mccallum, A. (n.d.). Optimizing Semantic Coherence in Topic Models.
- Mimno, D., Wallach, H. M., Talley, E., Leenders, M., & McCallum, A. (2011). Optimizing semantic coherence in topic models. In EMNLP 2011 - Conference on Empirical Methods in Natural Language Processing, Proceedings of the Conference. Association for Computational Linguistics.
- Ming, N. C. & Ming, V. L. (2012). Automated Predictive Assessment from Unstructured Student Writing. In Automated predcitive assessment from unstructured student writing (Issue c).
- Murillo, L. (2013). Cultura ambiental: un estudio desde las dimensiones de valor, creencias, actitudes y comportamientos ambientales. Producción + Limpia, 8(2), 94–105. http://search.ebscohost.com/login.aspx?direct=true&db=aph&AN=95426468&lang=es&site=ehost-live
- O’Callaghan, D., Greene, D., Carthy, J., & Cunningham, P. (2015). An analysis of the coherence of descriptors in topic modeling. Expert Systems with Applications, 42(13), 5645–5657. https://doi.org/10.1016/j.eswa.2015.02.055
- Prabhakaran, S. (2018). Gensim Topic Modeling - A Guide to Building Best LDA models. Machine Learning Plus. https://www.machinelearningplus.com/nlp/topic-modelinggensim-python/
- Ramadhan, S., Sukma, E., & Indriyani, V. (2019). Environmental education and disaster mitigation through language learning. IOP Conference Series: Earth and Environmental Science, 314(1), 012054. https://doi.org/10.1088/1755-1315/314/1/012054
- Röder, M., Both, A., & Hinneburg, A. (2015). Exploring the space of topic coherence measures. WSDM 2015 - Proceedings of the 8th ACM International Conference on Web Search and Data Mining, 399–408. https://doi.org/10.1145/2684822.2685324
- Sarkar, D. (2019). Text Analytics with Python. In Text Analytics with Python. https://doi.org/10.1007/978-1-4842-4354-1
- Seufert, S., Guggemos, J., & Sonderegger, S. (2019). Learning analytics in higher education using peer-feedback and self-assessment: Use case of an academic writing course. CSEDU 2019 - Proceedings of the 11th International Conference on Computer Supported
- Education, 2, 315–322. https://doi.org/10.5220/0007714603150322
- Song, Y., Pan, S., Liu, S., Zhou, M. X., & Qian, W. (2009). Topic and keyword re-ranking for LDA-based topic modeling. International Conference on Information and Knowledge Management, Proceedings, 1757–1760. https://doi.org/10.1145/1645953.1646223
- Stevens, K., Kegelmeyer, P., Andrzejewski, D., & Buttler, D. (2012). Exploring topic coherence over many models and many topics. In EMNLP-CoNLL 2012 - 2012 Joint Conference on Empirical Methods in Natural Language Processing and Computational Natural Language
- Learning, Proceedings of the Conference. Association for Computational Linguistics. http://mallet.cs.umass.edu/
- Topic Model Evaluation - High Demand Skills. (n.d.). Retrieved January 19, 2021, from https://highdemandskills.com/topic-model-evaluation/
- Topic modeling | Computing for the Social Sciences. (n.d.). Retrieved January 19, 2021, from https://cfss.uchicago.edu/notes/topic-modeling/
- Tran, B. X., Nghiem, S., Sahin, O., Vu, T. M., Ha, G. H., Vu, G. T., Pham, H. Q., Do, H. T., Latkin, C. A., Tam, W., Ho, C. S. H., & Ho, R. C. M. (2019). Modeling research topics for artificial intelligence applications in medicine: Latent dirichlet allocation application study.
- Journal of Medical Internet Research, 21(11), e15511. https://doi.org/10.2196/15511
- Valdez, D., Pickett, A. C., & Goodson, P. (2018). Topic Modeling: Latent Semantic Analysis for the Social Sciences. Social Science Quarterly, 99(5), 1665–1679. https://doi.org/10.1111/ssqu.12528
- Wang, T., & Liu, C. Y. (2017). JSEA: A Program Comprehension Tool Adopting LDA-based Topic Modeling. In IJACSA) International Journal of Advanced Computer Science and Applications (Vol. 8, Issue 3). https://github.com/jseaTool/JSEA
- Xing, W., Lee, H. S., & Shibani, A. (2020). Identifying patterns in students’ scientific argumentation: content analysis through text mining using Latent Dirichlet Allocation. Educational Technology Research and Development, 68(5), 2185–2214. https://doi.org/10.1007/s11423-020-09761-w
- Xun, G., Gopalakrishnan, V., Li, F. M. Y., Gao, J., & Zhang, A. (2017). Topic discovery for short texts using word embeddings. Proceedings - IEEE International Conference on Data Mining, ICDM, 1299–1304. https://doi.org/10.1109/ICDM.2016.33
- Yoshida, T., Hisano, R., & Ohnishi, T. (2020). Gaussian Hierarchical Latent Dirichlet Allocation: Bringing Polysemy Back. ArXiv. http://arxiv.org/abs/2002.10855
- Zheng, A. X., Cong, Z. X., Wang, J. R., Li, J., Yang, H. H., & Chen, G. N. (2013). Highlyefficient peroxidase-like catalytic activity of graphene dots for biosensing. Biosensors and Bioelectronics, 49, 519–524. https://doi.org/10.1016/j.bios.2013.05.038
- Zhu, G., Xing, W., Costa, S., Scardamalia, M., & Pei, B. (2019). Exploring emotional and cognitive dynamics of Knowledge Building in grades 1 and 2. User Modeling and UserAdapted Interaction, 29(4), 789–820. https://doi.org/10.1007/s11257-019-09241-8