Bootstrap versus Jackknife: Confidence intervals, hypothesis testing, density estimation, and kernel regression
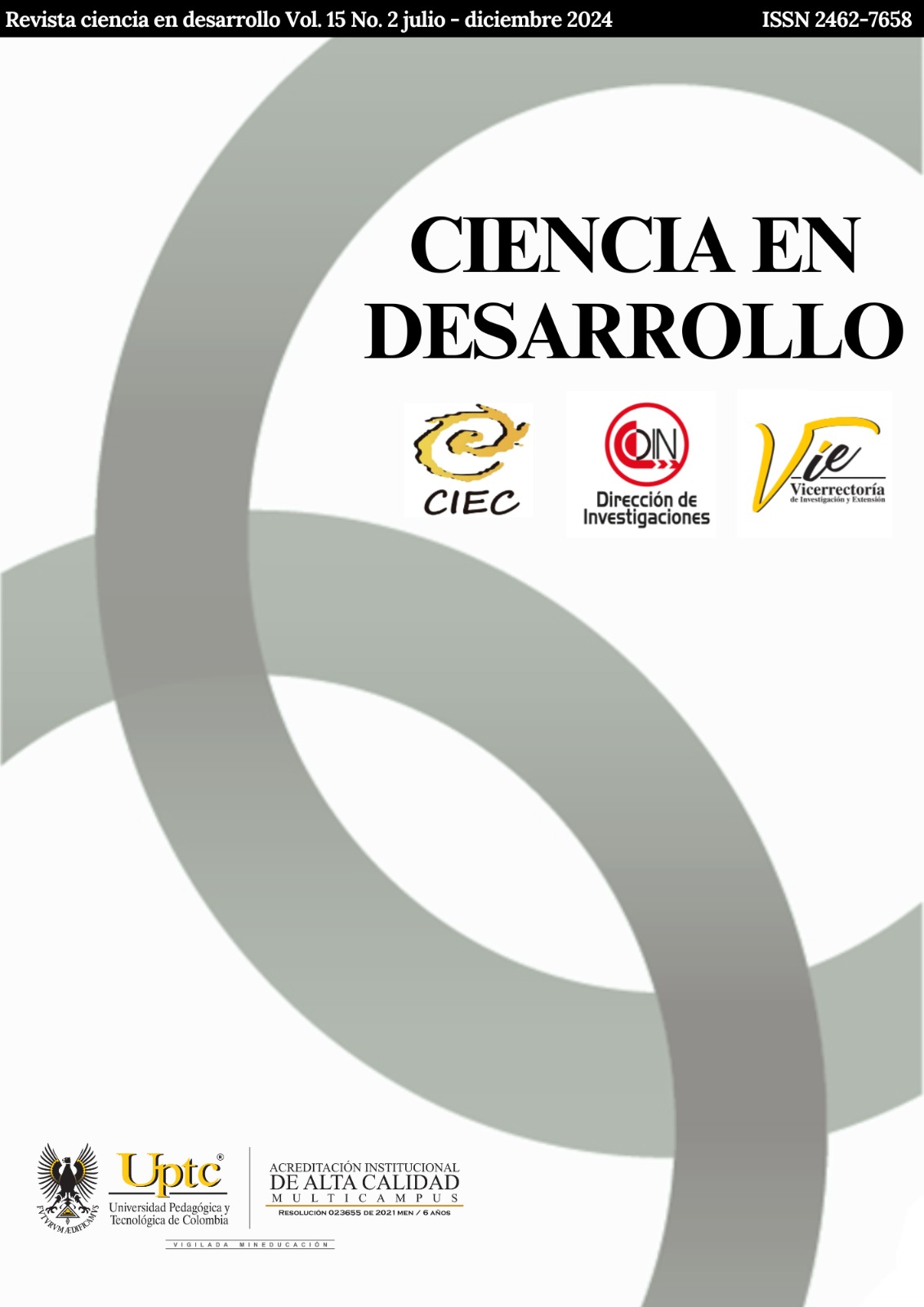
Resumen
Se comparan los métodos Bootstrap y Jackknife en varios contextos estadísticos. Inicialmente usando estimaciones de coeficientes de variación obtenidos a partir de muestras de varios modelos de probabilidad (Normal, Gama, Binomial y Poisson) generadas por simulación de Monte Carlo. Con los resultados se evalúa sesgo y varianza los estimadores. También se estudia el desempeño de los dos procedimientos inferenciales considerados en problemas de una muestra, estimación de la densidad y regresión kernel. Los resultados muestran que en el caso del coeficiente de variación Jackknife tiene menor sesgo pero mayor error estándar. Bootstrap es más potente en este contexto. En lo referente a la estimación de la densidad (histograma y Kernel) y la estimación del ancho de banda en la estimación de la función de regresión Jackknife produce estimaciones más cercanas a las clásicas que las halladas con Bootstrap. Los correspondientes intervalos de confianza con Jackknife son más cortos que los establecidos con Bootstrap.
Palabras clave
Bootstrap, estimación kernel de la densidad, intervalos de confianza, Jackknife, potencia de la prueba, regresión kernel
Citas
- Behme, A. Theory of Stochastic Objects: Probability, Stochastic Processes and Inference. Chapman &
- Hall/CRC Press, 2018
- Bonamente, M. Monte Carlo and Re-sampling Methods. In Statistics and Analysis of Scientific Data
- (pp. 351-367). Singapore: Springer Nature Singapore, 2022.
- Bose, A., Chatterjee, S. Comparison of bootstrap and jackknife variance estimators in linear regression:
- Second order results. Statistica Sinica. 2002, 575-598.
- Bowman, A., Azzalini, A. Applied smoothing techniques for data analysis: the kernel approach with
- S-Plus illustrations. OUP Oxford, 1973.
- Buckland, S. Monte Carlo confidence intervals. Biometrics. 1984; 811-817.
- Chen, Y. A tutorial on kernel density estimation and recent advances. Biostatistics & Epidemiology.
- ; 1(1), 161-187.
- DiCiccio, T., Efron, B. Bootstrap confidence intervals. Statistical Science. 1996; 11(3): 189-228.
- Donegani, M. Bootstrap adaptive test for two way analysis of variance. Biometrical Journal. 1992;
- (2): 141-146
- Donnelly-Makowecki, L., Moore, R. Hierarchical testing of three rainfall-runoff models in small
- forested catchments. Journal of Hydrology. 1999; 219(3-4): 136-152.
- Efron, B., Stein, C. The Jackknife estimate of variance. The Annals of Statistics. 1981; 586-596.
- Efron B., Tibshirani R. An introduction to the bootstrap. 1994; CRC Press.
- Freedman D., Peters S. Bootstrapping a regression equation: Some empirical results. Journal of Statistical
- Software. 2008; 25(7):1-37.
- Garay, A., Hashimoto, E., Ortega, E., Lachos, V. On estimation and influence diagnostics for zeroinflated
- negative binomial regression models. Computational Statistics & Data Analysis, 2011; 55(3):
- -1318.
- Gillibrand E., Jamieson A., Bagley, P., Zuur, A., Priede, I. Seasonal development of a deep pelagic
- bioluminescent layer in the temperate NE Atlantic Ocean. Marine Ecology Progress Series, 2007, 341:
- -43.
- Gong, G. Cross-validation, the jackknife, and the bootstrap: Excess error estimation in forward logistic
- regression. Journal of the American Statistical Association. 1986; 81 (393): 108-113.
- Hahn, J. Bootstrapping quantile regression estimators. Econometric Theory. 1995; 11(1): 105-121.
- Han, J., Li, G., Liu, H., Hu, H., Zhang, X. Stimulation of bioluminescence in Noctiluca sp. using
- controlled temperature changes. Luminescence. 2013; 28(5): 742-744.
- Heger, A., Ieno, E., King, N., Morris, K., Bagley, P., Priede, I. Deep-sea pelagic bioluminescence over
- the Mid-Atlantic Ridge. Deep Sea Research Part II: Topical Studies in Oceanography. 2008, 55(1-2),
- -136.
- Kohler, M., Schindler, A., Sperlich, S. 2014. A review and comparison of bandwidth selection methods
- for kernel regression. International Statistical Review. 2014; 82(2): 243-274.
- Lu, X., Su, L. Jackknife model averaging for quantile regressions. Journal of Econometrics. 2015;
- (1): 40-58.
- McCabe, P., Korb, O., Cole, J. Kernel density estimation applied to bond length, bond angle, and torsion
- angle distributions. Journal of Chemical Information and Modeling, 2014, 54(5): 1284-1288.
- Moulton, L., Zeger, S. Bootstrapping generalized linear models. Computational Statistics & Data
- Analysis. 1991; 11: 53-63.
- O´Brien, S., Webb, A., Brewer, M., Reid, J. Use of kernel density estimation and maximum curvature
- to set marine protected area boundaries: Identifying a special protection area for wintering red-throated
- divers in the UK. Biological Conservation. 2012, 156: 15-21.
- Oyeyemi, G. Comparison of bootstrap and jackknife methods of resampling in estimating popultion
- parameters. Global Journal of Pure and Applied Sciences. 2008; 1(2):217-220.
- Quenouille, M. Approximate test of correlation in time series. Journal of the Royal Statistical Society,
- Series B. 1949; 11:68-84
- Quenouille, M. Notes on bias in estimation. Biometrika. 1956; 43(3): 353-360.
- R Core Team. R: A Language and Environment for Statistical Computing. R Foundation for Statistical
- Computing. https://www.R-project.org/. 2022.
- Roda, A., Pasini, P., Mirasoli, M., Michelini, E., Guardigli, M. Biotechnological applications of
- bioluminescence and chemiluminescence. TRENDS in Biotechnology. 2004, 22(6), 295-303.
- Rudemo, M. Empirical choice of histograms and kernel density estimators. Scandinavian Journal of
- Statistics. 1982, 65-78.
- Scott, D. Histogram. Wiley Interdisciplinary Reviews: Computational Statistics. 2010, 2(1): 44-48.
- Sherman, M., Cessie, S. A comparison between bootstrap methods and generalized estimating equations
- for correlated outcomes in generalized linear models. Communications in Statistics-Simulation and
- Computation. 1997; 26(3): 901-925.
- Tukey, J. Bias and confidence in not quite large samples. Ann. Math. Statist. 1958; 29: 614.
- Widder, E. Marine bioluminescence. Why do so many animals in the open ocean make light?. Science.
- ; 328: 704-708.
- Wu, C. Jackknife, Bootstrap and other resampling methods in regression analysis. The Annals of
- Statistics. 1986; 14(4): 1261-1295.
- Zambom, A., Dias, R. A review of kernel density estimation with applications to econometrics. International
- Econometric Review. 2013; 5(1): 20-42.
- Zuur, A., Ieno, E. Smith, G. Analysing ecological data. Springer; 2007.
- Zuur, A. Additive mixed modelling applied on deep-sea pelagic bioluminescent organisms. In Mixed
- effects models and extensions in ecology with R. 2009; 399-422.