Proposal of a computational method for asbestos detection in hyperspectral images based on the identification of prominent peaks in the spectral signature
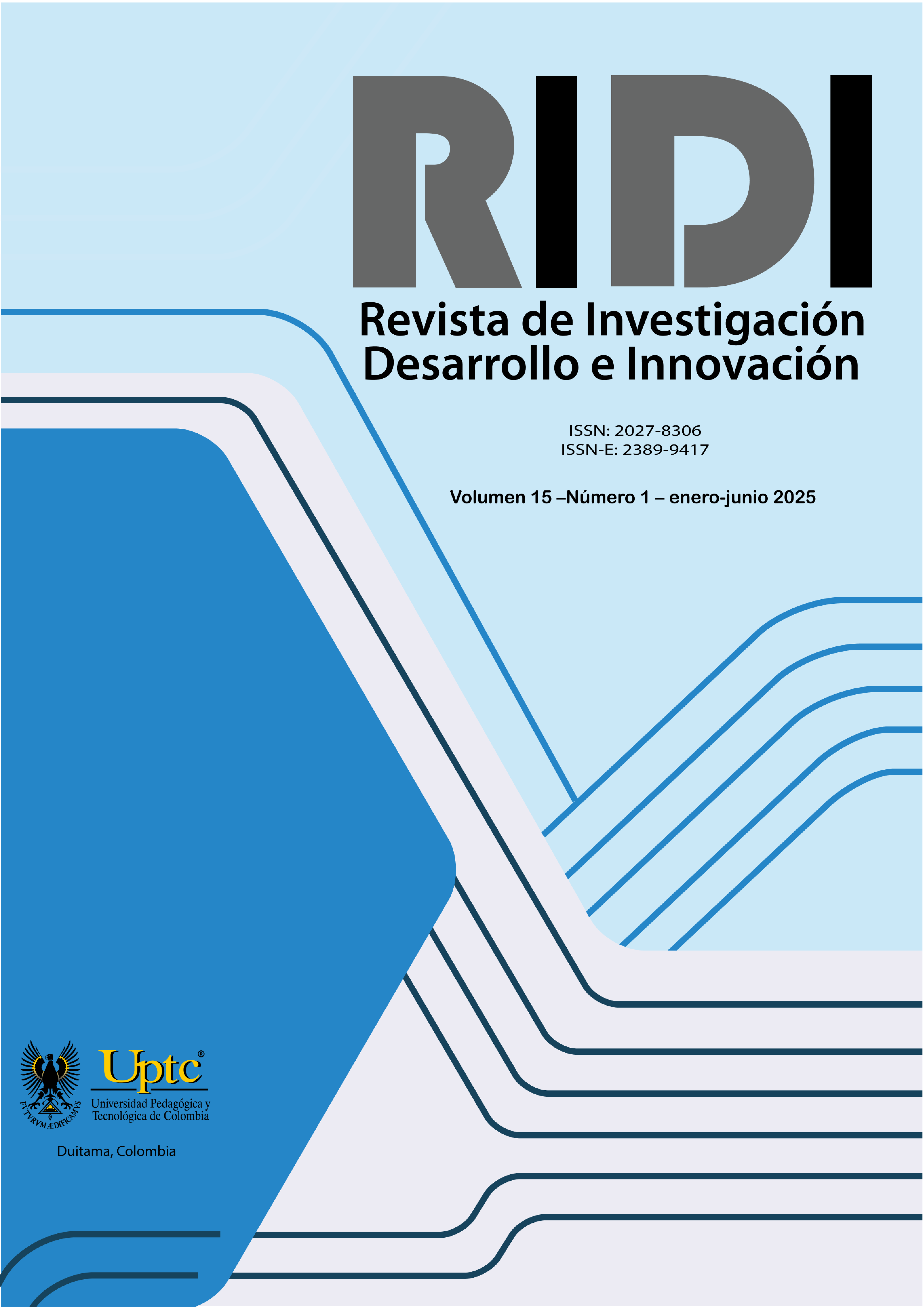
Abstract
This study proposes a computational method for asbestos detection in hyperspectral images. The methodology consists of five phases: selection of sample pixels and identification of the characteristic pixel, determination of prominent peaks in the spectral curve, method implementation with reference threshold definition, application to test images, and comparative evaluation of effectiveness and efficiency. The method identifies asbestos pixels by calculating the Euclidean distance between the prominent peaks of spectral curves. Results show no overlap between maximum distances of asbestos pixels and minimum distances of non-asbestos pixels, detecting 11.87% of asbestos pixels in the test image. Although the correlation method is 1.02% faster, the difference is negligible. This method can be extrapolated to other materials with similar spectral features, contributing to urban diagnostics of hazardous materials like asbestos.
Keywords
asbestos, hyperspectral imaging, maximum detection, remote sensing
Author Biography
Gabriel Elías Chanchí-Golondrino
Ingeniero en Electrónica y Telecomunicaciones de la Universidad del Cauca, Doctor en Ingeniería Telemática de la Universidad del Cauca.
Manuel Alejandro Ospina-Alarcón
Ingeniero de Control de la Universidad Nacional de Colombia, Doctor en Ingeniería - Ciencia y Tecnología de Materiales de la Universidad Nacional de Colombia.
Manuel Saba
Ingeniero Civil de la Universidad de Cagliari - Italia, Doctor en Ingeniería con énfasis en Ciencia y Tecnología de Materiales de la Universidad de Cartagena - Colombia.
References
- Abbasi, M., Mostafa, S., Vieira, A. S., Patorniti, N., & Stewart, R. A. (2022). Mapping Roofing with Asbestos-Containing Material by Using Remote Sensing Imagery and Machine Learning-Based Image Classification: A State-of-the-Art Review. Sustainability, 14 (13), 8068. https://doi.org/10.3390/su14138068
- Adão, T., Hruška, J., Pádua, L., Bessa, J., Peres, E., Morais, R., & Sousa, J. (2017). Hyperspectral Imaging: A Review on UAV-Based Sensors, Data Processing and Applications for Agriculture and Forestry. Remote Sensing, 9 (11), 1110. https://doi.org/10.3390/rs9111110
- Ai, W., Liu, S., Liao, H., Du, J., Cai, Y., Liao, C., Shi, H., Lin, Y., Junaid, M., Yue, X., & Wang, J. (2022). Application of hyperspectral imaging technology in the rapid identification of microplastics in farmland soil. Science of The Total Environment, 807, 151030. https://doi.org/10.1016/j.scitotenv.2021.151030
- Ang, K. L.-M., & Seng, J. K. P. (2021). Big Data and Machine Learning With Hyperspectral Information in Agriculture. IEEE Access, 9, 36699–36718. https://doi.org/10.1109/ACCESS.2021.3051196
- Bodkin, A., Sheinis, A., Norton, A., Daly, J., Beaven, S., & Weinheimer, J. (2009). Snapshot hyperspectral imaging: the hyperpixel array camera. In S. S. Shen & P. E. Lewis (Eds.), Algorithms and Technologies for Multispectral, Hyperspectral, and Ultraspectral Imagery XV, 73340H. https://doi.org/10.1117/12.818929
- Bonifazi, G., Capobianco, G., & Serranti, S. (2015). Hyperspectral imaging applied to the identification and classification of asbestos fibers. 2015 IEEE SENSORS, 1–4. https://doi.org/10.1109/ICSENS.2015.7370458
- Bonifazi, G., Capobianco, G., & Serranti, S. (2018). Asbestos containing materials detection and classification by the use of hyperspectral imaging. Journal of Hazardous Materials, 344, 981–993. https://doi.org/10.1016/j.jhazmat.2017.11.056
- Bonifazi, G., Capobianco, G., & Serranti, S. (2019). Hyperspectral Imaging and Hierarchical PLS-DA Applied to Asbestos Recognition in Construction and Demolition Waste. Applied Sciences, 9 (21), 4587. https://doi.org/10.3390/app9214587
- Bonifazi, G., Capobianco, G., Serranti, S., Trotta, O., Bellagamba, S., Malinconico, S., & Paglietti, F. (2024). Asbestos detection in construction and demolition waste by different classification methods applied to short-wave infrared hyperspectral images. Spectrochimica Acta Part A: Molecular and Biomolecular Spectroscopy, 307, 123672. https://doi.org/10.1016/j.saa.2023.123672
- Calvillo, E. A., Mendoza, R., Munoz, J., Martinez, J. C., Vargas, M., & Rodriguez, L. C. (2016). Automatic algorithm to classify and locate research papers using natural language. IEEE Latin America Transactions, 14 (3), 1367–1371. https://doi.org/10.1109/TLA.2016.7459622
- Ding, X., Zhang, S., & Li, H. (2016). A Polymorphic Ant Colony Algorithm (PACA) for the Selection of Optimized Band Selection of Hyperspectral Remote Sensing Image. Proceedings of the 2016 International Conference on Engineering and Technology Innovations. https://doi.org/10.2991/iceti-16.2016.37
- Eckhard, J., Eckhard, T., Valero, E. M., Nieves, J. L., & Contreras, E. G. (2015). Outdoor scene reflectance measurements using a Bragg-grating-based hyperspectral imager. Applied Optics, 54 (13), D15. https://doi.org/10.1364/AO.54.000D15
- Eismann, M. T. (2012). Hyperspectral Remote Sensing. SPIE. https://doi.org/10.1117/3.899758
- Espinosa-Zúñiga, J. J. (2020). Aplicación de metodología CRISP-DM para segmentación geográfica de una base de datos pública. Ingeniería, Investigación y Tecnología, 21 (1), 1–13. https://doi.org/10.22201/fi.25940732e.2020.21n1.008
- Frassy, F., Candiani, G., Rusmini, M., Maianti, P., Marchesi, A., Nodari, F., Via, G., Albonico, C., & Gianinetto, M. (2014). Mapping Asbestos-Cement Roofing with Hyperspectral Remote Sensing over a Large Mountain Region of the Italian Western Alps. Sensors, 14 (9), 15900–15913. https://doi.org/10.3390/s140915900
- Gao, L., & Smith, R. T. (2015). Optical hyperspectral imaging in microscopy and spectroscopy - a review of data acquisition. Journal of Biophotonics, 8 (6), 441–456. https://doi.org/10.1002/jbio.201400051
- Gao, L., Yao, D., Li, Q., Zhuang, L., Zhang, B., & Bioucas-Dias, J. (2017). A New Low-Rank Representation Based Hyperspectral Image Denoising Method for Mineral Mapping. Remote Sensing, 9 (11), 1145. https://doi.org/10.3390/rs9111145
- González-Núñez, H., & de la Fuente, R. (2017). Offner imaging spectrometers. Optica Pura y Aplicada, 50 (1), 37–47. https://doi.org/10.7149/OPA.50.1.49506
- González, C., Resano, J., Mozos, D., Plaza, A., & Valencia, D. (2010). FPGA Implementation of the Pixel Purity Index Algorithm for Remotely Sensed Hyperspectral Image Analysis. EURASIP Journal on Advances in Signal Processing, 2010 (1), 969806. https://doi.org/10.1155/2010/969806
- Gu, Y., Liu, T., Gao, G., Ren, G., Ma, Y., Chanussot, J., & Jia, X. (2021). Multimodal hyperspectral remote sensing: an overview and perspective. Science China Information Sciences, 64 (2), 121301. https://doi.org/10.1007/s11432-020-3084-1
- Islam, T., Islam, R., Uddin, P., & Ulhaq, A. (2024). Spectrally Segmented-Enhanced Neural Network for Precise Land Cover Object Classification in Hyperspectral Imagery. Remote Sensing, 16 (5), 807. https://doi.org/10.3390/rs16050807
- Kaplan, G., Gašparović, M., Kaplan, O., Adjiski, V., Comert, R., & Mobariz, M. A. (2023). Machine Learning-Based Classification of Asbestos-Containing Roofs Using Airborne RGB and Thermal Imagery. Sustainability, 15 (7), 6067. https://doi.org/10.3390/su15076067
- Kapre, A., Kunde, S., Mittal, S., & Singhal, R. (2023). RAxC: Reflexivity-based Approximate Computing techniques for efficient remote sensing. 2023 IEEE International Conference on Big Data (BigData), 1168–1173. https://doi.org/10.1109/BigData59044.2023.10386511
- Khan, M. J., Khan, H. S., Yousaf, A., Khurshid, K., & Abbas, A. (2018). Modern Trends in Hyperspectral Image Analysis: A Review. IEEE Access, 6, 14118–14129. https://doi.org/10.1109/ACCESS.2018.2812999
- Lim, H.-T., & Murukeshan, V. M. (2017). Spatial calibration and image processing requirements of an image fiber bundle based snapshot hyperspectral imaging probe: from raw data to datacube. In A. K. Asundi (Ed.), Fifth International Conference on Optical and Photonics Engineering, 104491P. https://doi.org/10.1117/12.2270739
- Lu, B., Dao, P., Liu, J., He, Y., & Shang, J. (2020). Recent Advances of Hyperspectral Imaging Technology and Applications in Agriculture. Remote Sensing, 12 (16), 2659. https://doi.org/10.3390/rs12162659
- Martinez-Plumed, F., Contreras-Ochando, L., Ferri, C., Hernandez-Orallo, J., Kull, M., Lachiche, N., Ramirez-Quintana, M. J., & Flach, P. (2021). CRISP-DM Twenty Years Later: From Data Mining Processes to Data Science Trajectories. IEEE Transactions on Knowledge and Data Engineering, 33 (8), 3048–3061. https://doi.org/10.1109/TKDE.2019.2962680
- Mendes, V. B., Leta, F. R., Conci, A., & Gonçalves, L. B. (2010). Detección de Posición Angular de Embarcaciones, utilizando Técnicas de Visión Computacional y Redes Neurales Artificiales. Información Tecnológica, 21 (6). https://doi.org/10.4067/S0718-07642010000600018
- Moroni, M., Lupo, E., Marra, E., & Cenedese, A. (2013). Hyperspectral Image Analysis in Environmental Monitoring: Setup of a New Tunable Filter Platform. Procedia Environmental Sciences, 19, 885–894. https://doi.org/10.1016/j.proenv.2013.06.098
- Padhan, P. C., & others. (2012). Application of ARIMA model for forecasting agricultural productivity in India. Journal of Agriculture and Social Sciences, 8 (2), 50–56.
- Paoletti, M. E., Haut, J. M., Plaza, J., & Plaza, A. (2019). Estudio Comparativo de Técnicas de Clasificación de Imágenes Hiperespectrales. Revista Iberoamericana de Automática e Informática Industrial, 16 (2), 129. https://doi.org/10.4995/riai.2019.11078
- Park, B. (2016). Future Trends in Hyperspectral Imaging. NIR News, 27 (1), 35–38. https://doi.org/10.1255/nirn.1583
- Pérez-Roncal, C., López-Maestresalas, A., López-Molina, C., Marín-Ederra, D., Urrestarazu-Vidart, J., Arazuri-Garín, S., Santesteban-García, L. G., & Jarén-Ceballos, C. (2019). Identificación de la presencia de oídio (Erysiphe necator) en racimos de uva mediante imágenes hiperespectrales. Congreso Ibérico de Agroingeniería, 1248–1254. https://doi.org/10.26754/c_agroing.2019.com.3434
- Peyghambari, S., & Zhang, Y. (2021). Hyperspectral remote sensing in lithological mapping, mineral exploration, and environmental geology: an updated review. Journal of Applied Remote Sensing, 15 (03). https://doi.org/10.1117/1.JRS.15.031501
- Sahoo, R. (2020). Hyperspectral Remote Sensing. Elsevier. https://doi.org/10.1016/C2018-0-01850-2
- Tan, K., Wang, H., Chen, L., Du, Q., Du, P., & Pan, C. (2020). Estimation of the spatial distribution of heavy metal in agricultural soils using airborne hyperspectral imaging and random forest. Journal of Hazardous Materials, 382, 120987. https://doi.org/10.1016/j.jhazmat.2019.120987
- Valdelamar-Martínez, D., Saba, M., & Torres-Gil, L. K. (2024). Assessment of asbestos-cement roof distribution and prioritized intervention approaches through hyperspectral imaging. Heliyon, 10 (3), e25612. https://doi.org/10.1016/j.heliyon.2024.e25612
- Wang, C., Liu, B., Liu, L., Zhu, Y., Hou, J., Liu, P., & Li, X. (2021). A review of deep learning used in the hyperspectral image analysis for agriculture. Artificial Intelligence Review, 54 (7), 5205–5253. https://doi.org/10.1007/s10462-021-10018-y
- Yi, L., Chen, J. M., Zhang, G., Xu, X., Ming, X., & Guo, W. (2021). Seamless Mosaicking of UAV-Based Push-Broom Hyperspectral Images for Environment Monitoring. Remote Sensing, 13 (22), 4720. https://doi.org/10.3390/rs13224720
- Zhang, L., & Zhong, Y. (2010). Analysis of Hyperspectral Remote Sensing Images. In Geospatial Technology for Earth Observation, 235–269. Springer US. https://doi.org/10.1007/978-1-4419-0050-0_9
- Zhang, X., Wang, Y., Zhang, N., Xu, D., Luo, H., Chen, B., & Ben, G. (2020). SSDANet: Spectral-Spatial Three-Dimensional Convolutional Neural Network for Hyperspectral Image Classification. IEEE Access, 8, 127167–127180. https://doi.org/10.1109/ACCESS.2020.3008029fgjgh