Penalized Bayesian D-Optimal Designs for Regression Models of Continuous Response
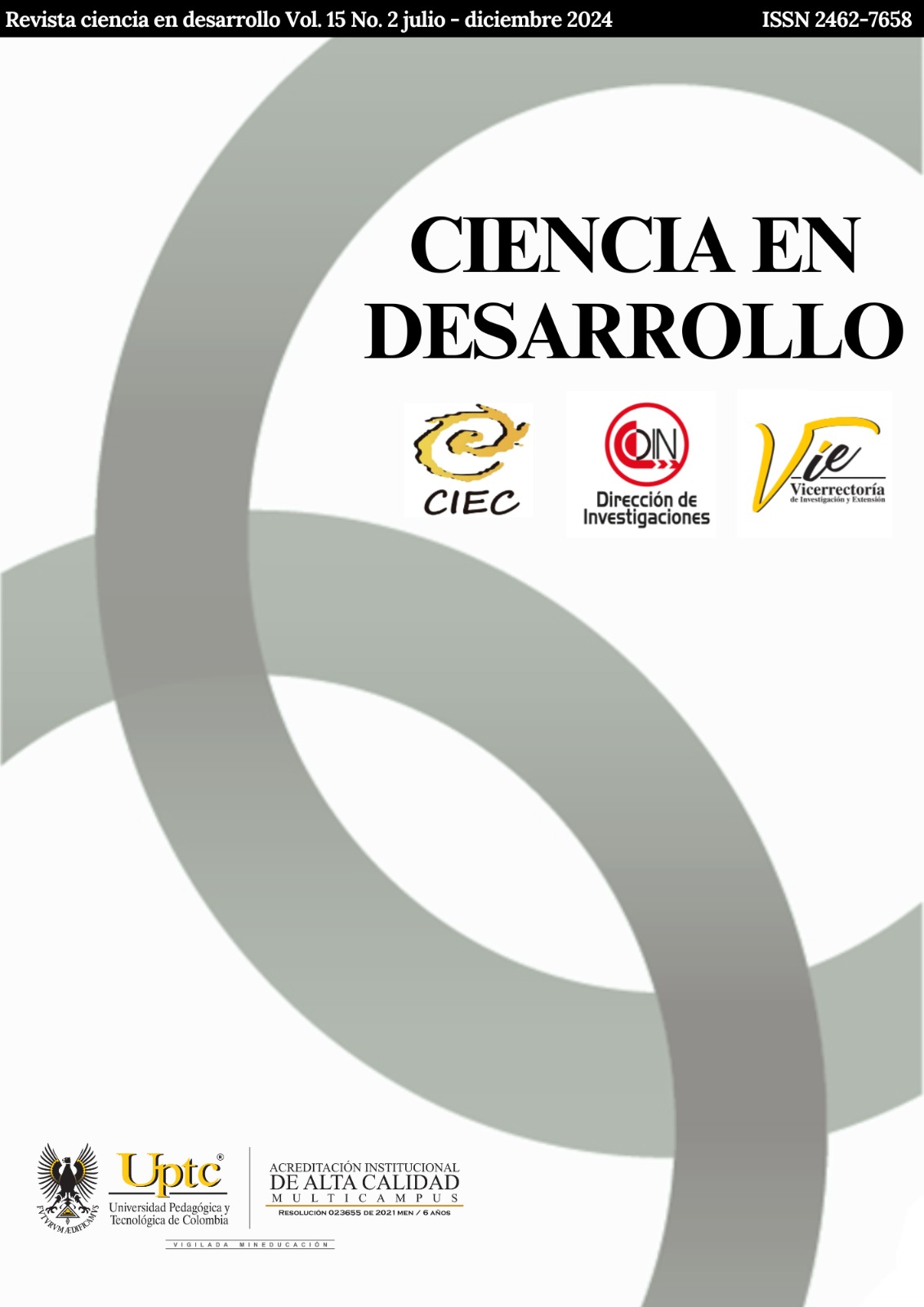
Abstract
We propose extending the use of desirability functions in Bayesian optimal designs for regression models. This technique generates experimental designs with good statistical inference properties according to Bayesian optimal design theory and practical features, as defined by an investigator. These practical features are defined by a penalty function, using an overall desirability function, which is added to a Bayesian $D$-optimal design criterion to penalize impractical experimental designs. This methodology is illustrated by two examples of regression models: quadratic and exponential. Then, we compare designs obtained for different prior distributions of unknown parameters by efficiency calculations and simulation study. Results show that the $D$-efficiencies of the penalized designs relative to the non-penalized Bayesian D-optimal designs are competitive.
Keywords
Bayesian Optimal Designs, Desirability Functions, Exponential Growth Model, Penalized Designs, Quadratic Regression Model
References
- H. Chernoff, “Locally Optimal Designs for Estimating Parameters”, The Annals of Mathematical Statistics, vol. 24, no. 4, pp. 586-602,
- K. Chaloner and I. Verdinelli, “Bayesian experimental design: A review”, Statistical Science, vol. 10, no. 3, pp. 273-304, 1995.
- G. E. Box and N. R. Draper, “Robust designs”,Biometrika, vol. 62, no. 2, pp. 347-352, 1975.
- V. Dragalin and V. Fedorov, “Adaptive designs for dose-finding based on efficacy-toxicity response”, Journal of Statistical Planning and Inference, vol. 136, no. 6, pp. 1800-1823, 2006.
- V. Dragalin, V. Fedorov and Y. Wu, “Adaptive designs for selecting drug combinations based on efficacy-toxicity response”, Journal of Statistical Planning and Inference, vol. 138, no. 2, pp. 352-373, 2008.
- L. Pronzato, “Penalized optimal designs for dose-finding”, Journal of Statistical Planning and Inference, vol. 140, no. 1, pp. 283-296,
- S. M. Parker and C. Gennings, “Penalized locally optimal experimental designs for nonlinear models”, Journal of agricultural, biological, and environmental statistics, vol. 13, no. 3, pp. 334-354, 2008.
- F.-C. Wu, “Optimization of correlated multiple quality characteristics using desirability function”, Quality Engineering, vol. 17, no. 1, pp. 119-126, 2004.
- E. C. Harrington, “The desirability function”, Industrial quality control, vol. 21, no. 10, pp. 494-498, 1965.
- S. I. Rudnykh y V. I. López Ríos, “Elección de la función de deseabilidad para diseños óptimos bajo restricciones”, Revista EIA, vol. 15, no. 30, pp. 13-24, 2018.
- M. Shih, C. Gennings, V.M. Chinchilli and W.H Carter Jr, “Titrating and evaluating multidrug regimens within subjects”, Statistics in
- medicine, vol. 22, no. 14, pp. 2257-2279, 2003.
- A. DasGupta and W. Studden, “Robust bayesian experimental designs in normal linear models”, The Annals of Statistics, vol. 19, no. 3, pp. 1244-1256, 1991.
- J. A. Nelder and R. Mead, “A simplex method for function minimization”, The computer journal, vol. 7, no. 4, pp. 308-313, 1965.
- J. Pilz, “Bayesian estimation and experimental design in linear regression models”. JohnWiley & Sons Inc, NY, 1991.
- R. D. Gibb, “Optimal treatment combination estimation for univariate and multivariate response surface applications”. Ph.D. thesis Virginia Commonwealth University, Richmond, Virginia, USA, 1998.
- R Core Team “R: A language and environment for statistical computing”. R foundation for statistical computing, Vienna, Austria, 2021, https://www.r-project.org/
- S. M. Ermakov and A. A. Zhiglijavsky, “Matematitscheskaja teorija optimalnich experimentov”. Nauka, Moskva, 1987.
- V. V. Fedorov and S. L. Leonov, “Optimal design for nonlinear response models”. CRC Press, 2013.
- H. Dette and S. Sperlich, “A Note on Bayesian D-optimal Designs for a Generalization of the Exponential Growth Model”, South African Statistical Journal, vol. 28, pp. 103-117, 1994.
- D. Firth and J. Hinde, “On Bayesian Doptimum Design Criteria and the Equivalence Theorem in Non-linear Models.”, Journal of the Royal Statistical Society: Series B (Statistical Methodology), vol. 59, no. 4, pp. 793-797, 1997.
- B. P. Duarte and W. K. Wong, “Finding Bayesian Optimal Designs for Nonlinear Models: A Semidefinite Programming-Based Approach”, International Statistical Review, vol. 83, no. 2, pp. 239-262, 2015.
- S. Mukhopadhyay and L. M. Haines, “Bayesian D-optimal Designs for the Exponential Growth Model.”, Journal of Statistical Planning and Inference, vol. 44, no. 3, pp. 385-397, 1995.
- S. I. Rudnykh, “Penalized bayesian optimal designs for nonlinear models of Continuous Response”. Ph.D. thesis Universidad Nacional de Colombia, Medellín, Colombia, 2020.