Design Of A Correlational Model To Quantify Physicochemical Variables From Spectroradiometry Techniques In Water Bodies. Case Study Cuja River Cundinamarca Colombia
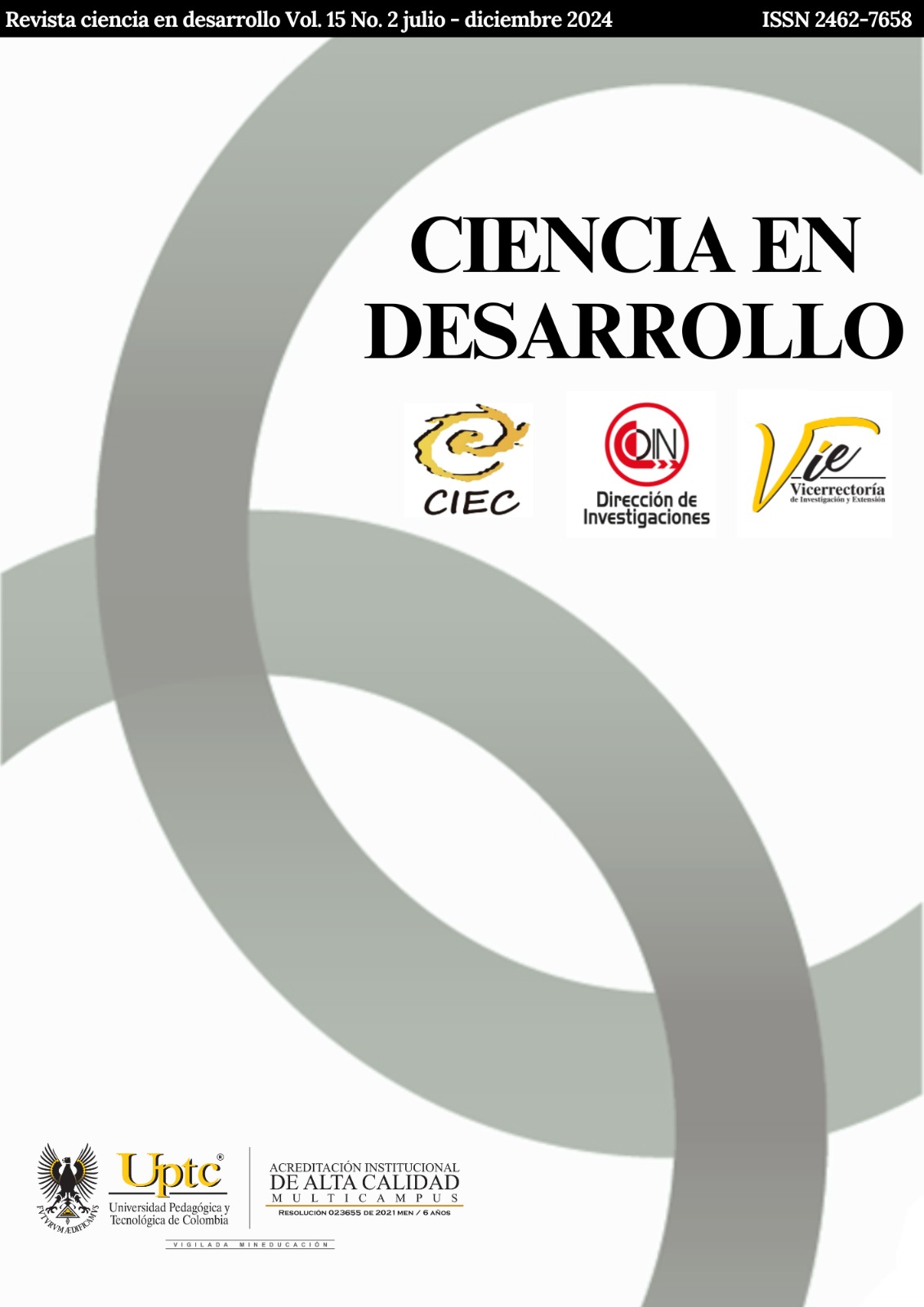
Abstract
Water quality is a critical issue in environmental management, and in this sense, remote sensing has acquired a relevant role as a highly effective evaluation tool. With its ability to obtain data remotely and on a large scale, remote sensing is presented as an innovative and reliable solution to monitor and analyze contamination in freshwater bodies in an increasingly accurate and timely manner. In this research, the correlation between physicochemical variables and data obtained by field spectro-radiometry was analyzed. The variables were conductivity, pH, total suspended solids (TSS), chemical oxygen demand (COD), nitrates and phosphates, taken at four different points in the Cuja river basin. 70 spectral signatures were captured, using the ASD FieldSpec HandHeld 2 handheld spectrum radiometer. Using the Pearson correlation coefficient and the R-squared coefficient of determination, the input data were analyzed in regression models with a confidence level of 95 %, showing a strong correlation between the pH variables of the water with a determination level of 92% and the wavelength of the visible spectrum of 400 nm. Likewise, it was determined that the 822 nm wavelength in the infrared range is highly effective for measuring nitrate levels with a determination coefficient of 90%, while the 760 nm wavelengths of the red edge and 393nm of the visible blue were adequate to measure phosphates and suspended solids with coefficients of 86% and 82%, respectively. Notably, in comparison, the conductivity and chemical oxygen demand (COD) variables exhibited coefficients of determination of 82% and 77%, respectively. These findings suggest that field spectroradiometry is a valuable ally in the measurement of physicochemical parameters in water quality.
Keywords
Remote sensing, spectroradiometry, physicochemical variables, water quality.
Author Biography
Edier Fernando Avila Velez
Bogota
References
- A.Dourojeanni, A. Jouravlev, CEPAL Gestión de cuencas y ríos vinculados con centros urbanos [online]. CEPAL. 1999.Disponible en: https://bibliotecadigital.ciren.cl/handle/20.500.13082/33031
- P.R. Kannel, S. Lee, S.R. Kanel, “Spatial–temporal variation and comparative assessment of water qualities of urban river system: a case study of the river Bagmati (Nepal)”, Environ Monit Assess, vol. 129, pp. 433–45, january 2007. https://doi.org/10.1007/s10661-006-9375-6
- Ministerio de Ambiente, Vivienda y Desarrollo Territorial, Política Nacional para la Gestión Integral del Recurso Hídrico[online]. Bogotá, D.C.: Colombia, 2010 Disponible en: https://www.minambiente.gov.co/wp-content/uploads/2021/10/Politica-nacional-Gestion-integral-de-recurso-Hidrico-web.pdf
- M.H. Gholizadeh, A.M. Melesse, L.A. Reddi, “Comprehensive Review on Water Quality Parameters E stimation Using Remote Sensing Techniques”, Sensors vol. 16, pp. 12-98, agosto 2016. https://doi.org/10.3390/s16081298
- N.E. Samboni, Y. Carvajal, J.C. Escobar, “Revisión de parámetros fisicoquímicos como indicadores de calidad y contaminación del agua” Ingeniería e investigación vol 27 no 3, pp. 172-181, diciembre 2007. https://www.redalyc.org/articulo.oa?id=64327320
- B.A. Schaeffer, K.G. Schaeffer, D. Keith, R.S. Lunetta, R. Conmy, R.W. Gould, “Barriers to adopting satellite remote sensing for water quality management”,Int J Remote Sens, vol.34, pp. 7534-7544, Agosto 2013. https://doi.org/10.1080/01431161.2013.82354
- R.H. Becker, M. Sayers, D. Dehm, R. Shuchman, K. Quintero, K. Bosse, R. Sawtell, " Unmanned aerial system based spectroradiometer for monitoring harmful algal blooms: A new paradigm in water quality monitoring", Journal of Great Lakes Research, vol. 45 no 3, pp.444-53, june 2019. https://doi.org/10.1016/J.JGLR.2019.03.006
- Y. Zhang, X. Kong, L. Deng, Y. Liu, Y. " Monitor water quality through retrieving water quality parameters from hyperspectral images using graph convolution network with superposition of multi-point effect: A case study in Maozhou River", Journal of Environmental Management, vol. 342, pp. 118283, september 2023. https://doi.org/10.1016/J.JENVMAN.2023.118283
- S. Chen, L. Han, X. Chen, D. Li, L. Sun, Y. Li, " Estimating wide range Total Suspended Solids concentrations from MODIS 250-m imageries: An improved method. ISPRS", Journal of Photogrammetry and Remote Sensing, vol. 99, pp. 58–69, january 2015. https://doi.org/10.1016/J.ISPRSJPRS.2014.10.006
- S. Imen, N. Chang, Bin, Y.J. Yang, " Developing the remote sensing-based early warning system for monitoring TSS concentrations in Lake Mead". Journal of Environmental Management, vol. 160, pp. 73–89, september 2015. https://doi.org/10.1016/J.JENVMAN.2015.06.003
- S. Chen, L. Fang, L. Zhang, W. Huang, " Remote sensing of turbidity in seawater intrusion reaches of Pearl River Estuary – A case study in Modaomen water way, China",. Estuarine, Coastal and Shelf Science, vol. 82 no1, pp. 119–127, march 2009. https://doi.org/10.1016/J.ECSS.2009.01.003
- S.H. Rahat, T. Steissberg, W. Chang, X. Chen, G. Mandavya, J. Tracy, A. Wasti, G. Atreya, S. Saki, M.A. Bhuiyan, P. Ray, "Remote sensing-enabled machine learning for river water quality modeling under multidimensional uncertainty", Science of The Total Environment, vol. 898, pp. 165-504, noviembre 2023. https://doi.org/10.1016/J.SCITOTENV.2023.165504
- M.G. Uddin, S. Nash, A. Rahman, A.I. Olbert, " A comprehensive method for improvement of water quality index (WQI) models for coastal water quality assessment", Water Research, vol 219, 118532, july 2022. https://doi.org/10.1016/J.WATRES.2022.118532
- M.L. Wu, Y.S. Wang, Y.T. Wang, J.P. Yin, J. Dong, Z.Y. Jiang, F.L. Sun, " Scenarios of nutrient alterations and responses of phytoplankton in a changing Daya Bay, South China Sea". Journal of Marine Systems, vol. 165, pp. 1–12, january 2017). https://doi.org/10.1016/J.JMARSYS.2016.09.004
- R. Beck, S. Zhan, H. Liu, S. Tong, B. Yang, M. Xu, Z. Ye, Y. Huang, S. Shu, Q. Wu, S. Wang, K. Berling, A. Murray, E. Emery, M. Reif, J. Harwood, J. Young, C. Nietch, D. Macke, H. Su, " Comparison of satellite reflectance algorithms for estimating chlorophyll-a in a temperate reservoir using coincident hyperspectral aircraft imagery and dense coincident surface observations",. Remote Sensing of Environment, vol. 178, pp. 15–30, june 2016. https://doi.org/10.1016/J.RSE.2016.03.002
- J. Cai, L. Meng, H. Liu, J. Chen, Q Xing, " Estimating Chemical Oxygen Demand in estuarine urban rivers using unmanned aerial vehicle hyperspectral images", Ecological Indicators, vol. 139, 108936, june 2022. https://doi.org/10.1016/J.ECOLIND.2022.108936
- Y. Qiu, H.E. Zhang, X. Tong, Y. Zhang, J. Zhao, Monitoring the water quality of water resources reservation area in upper region of Huangpu River using remote sensing[online]. IEEE International Symposium on Geoscience and Remote Sensing pp. 1082-1085, 2006 Disponible en: 10.1109/IGARSS.2006.279.
- X. Cai, Y. Li, S. Lei, S. Zeng, Z. Zhao, H. Lyu, X. Dong, J. Li, H. Wang, J. Xu, Y. Zhu, L. Wu, X. Cheng, "A hybrid remote sensing approach for estimating chemical oxygen demand concentration in optically complex waters: A case study in inland lake waters in eastern China". Science of The Total Environment, vol. 856, 158869. january 2023. https://doi.org/10.1016/J.SCITOTENV.2022.158869
- S.H. Rahat, Y. Steissberg, W. Chang, X. Chen, G. Mandavya, J. Tracy, A. Wasti, G. Atreya, S. Saki, M.A. Bhuiyan, P. Ray, “Remote sensing-enabled machine learning for river water quality modeling under multidimensional uncertainty”, Science of The Total Environment, vol. 898, 165504, 2023 https://doi.org/10.1016/J.SCITOTENV.2023.165504
- M.G. Uddin, S. Nash, A. Rahman, A.I. Olbert, “A comprehensive method for improvement of water quality index (WQI) models for coastal water quality assessment”. Water Research, vol. 219, 118532, 2022. https://doi.org/10.1016/J.WATRES.2022.118532
- C. Zhou, J. Zhang, "Simultaneous measurement of chemical oxygen demand and turbidity in water based on broad optical spectra using backpropagation neural network". Chemometrics and Intelligent Laboratory Systems, vol. 237, 104830, 202. https://doi.org/10.1016/J.CHEMOLAB.2023.104830
- R. Chafloque, C. Rodriguez, Y. Pomachagua, M. Hilario, M. “Predictive Neural Networks Model for Detection of Water Quality for Human ConsumptionIn 2021” 13th International Conference on Computational Intelligence and Communication Networks (CICN), pp. 172-176, september 2021.
- A.H. Haghiabi, A.H. Nasrolahi, A. Parsaie, “Water quality prediction using machine learning methods Water Quality”, Revista de investigación sobre la calidad del agua vol.53 no 1 pp. 3-13, 2018. https://doi.org/10.2166/wqrj.2018.025
- A.C. Aguilar, F.F. Obando, “APRENDIZAJE AUTOMÁTICO PARA LA PREDICCIÓN DE CALIDAD DE AGUA POTABLE”, INGENIARE, vol. 28, pp. 59–74, 2020. https://doi.org/https://doi.org/10.18041/1909-2458/ingeniare.28.6215
- M.F. Gutiérrez Fonseca, Modelo de gobernanza del agua en la Cuenca del Río Cuja [online]. Universidad UDCA, 2018 Disponible en:
- https://repository.udca.edu.co/handle/11158/963.
- Corporación Autónoma Regional CAR, Delimitación y localización de la cuenca Sumapaz y subcuenca río alto Sumapaz. En: CAR. Diagnóstico, prospectiva y formulación de la cuenca hidrográfica del río Sumapaz. Plan de Manejo y Ordenamiento de la Cuenca (POMCA) del río Sumapaz [online], Informe POMCA-002 UT. Bogotá DC: ed. CAR; 2016 Disponible en: https://www.car.gov.co/uploads/files/5ac68e4163931.pdf
- P. Chober, L.A. Schwarte, “Correlation coefficients: Appropriate use and interpretation”. Anesthesia and Analgesia, vol. 126, no 5, pp. 1763–1768, 2018. https://doi.org/10.1213/ANE.0000000000002864
- J.L. Rodgers, W.A NiceWander, “Thirteen ways to look at the correlation coefficient”, American Statistician, vol. 42, No 1, pp. 59–66, 1988. https://doi.org/10.1080/00031305.1988.10475524
- D. Chicco, M.J. Warrens, G. Jurman, “The coefficient of determination R-squared is more informative than SMAPE, MAE, MAPE, MSE and RMSE in regression analysis evaluation. PeerJ” Computer Science, vol. 7, pp. 1–24, 2021. https://doi.org/10.7717/PEERJ-CS.623
- J.D. Nagelkerke, “Miscellanea A note on a general definition of the coefficient of determination” Biometrika, Vol. 78, No 3, pp. 691-692, september, 1991. https://academic.oup.com/biomet/article-abstract/78/3/691/256225?redirectedFrom=fulltext&login=false
- J.L. Wu, C.R. Ho, C.C., Huang, A.L. Srivastav, J.H. Tzeng, Y.T. Lin, “Hyperspectral sensing for turbid water quality monitoring in freshwater rivers: Empirical relationship between reflectance and turbidity and total solids”. Sensors (Switzerland), vol. 14, No 12, pp. 22670–22688, 2014. https://doi.org/10.3390/s141222670.
- I. Won Seo, H. Yun, S.Y. Choi, “Forecasting Water Quality Parameters by ANN Model using Pre-processing Technique at The Downstream of Cheongpyeong Dam. Procedi” Engineering, vol, 154, pp. 1110–1115, 2016. https://doi.org/10.1016/j.proeng.2016.07.519
- I. Melendez Pastor, J. Navarro Pedreño, I. Gómez, M. Koch, “Identifying optimal spectral bands to assess soil properties with VNIR radiometry in semi-arid soils”. Geoderma, vol. 147, No 3–4, pp. 126–132, 2008. https://doi.org/10.1016/j.geoderma.2008.08.004
- J.D. Aguilar Sánchez, N. Cubas Irigoín, N. “Contaminación de suelos por el uso de aguas residuales”, Revista Alfa, vol. 5, No 14, pp. 132–144, 2021. https://doi.org/10.33996/revistaalfa.v5i14.104
- J.W. Lopes, F.B. Lopes, E.M. Andrade, L.C.G. Chaves, M.G.R.Carneiro, “Spectral Response of Water Under Different Concentrations of Suspended Sediment: Measurement and Simplified Modeling”, Journal of Agricultural Science, vol. 11, No 3, pp. 327. 2019. https://doi.org/10.5539/jas.v11n3p327
- S. Hafeez, M.S. Wong, S. Abbas, Y.T. Kwok, J. Nichol. K.H. Lee, D. Tang, L. Pun, “Detection and Monitoring of Marine Pollution Using Remote”, Sensing Technologies, 2018. https://books.google.com.co/books?hl=es&lr=&id=mTj8DwAAQBAJ&oi=fnd&pg=PA7&ots=Om7DvOxZnY&sig=wbnlh9UuyuqkcMaUIjQpI8k7b3s&redir_esc=y#v=onepage&q&f=false
- C. De La Hoz, C. Gotilla, MODELO PARA LA PREDICCION DE PARAMETROS DE CALIDAD DEL AGUA DEL LAGO DE VALENCIA UTILIZANDO IMÁGENES DE SATELITE [online], XIII Congreso de la Asociación Españolade teledetección 2009 Disponible en: http://www.aet.org.es/congresos/xiii/cal29.pdf
- J. Gallego Ayala, J. A. Gómez Limón, “Análisis de instrumentos políticos para el control de la contaminación por nitratos de la agricultura de regadío en Castilla y León (España)”. Spanish Journal of Agricultural Research, vol. 7, No 1, pp. 24–40, 2009, https://doi.org/10.5424/sjar/2009071-395
- D. Wang, Q. Cui, F. Gong, L. Wang, X. He, Y. Bai, “Satellite retrieval of Surface wáter nutrients in the coastal regions of the east China Sea”. Remote Sensing, vol. 10, No 12, pp. 1896, noviembre, 2018. https://www.mdpi.com/2072-4292/10/12/1896
- Q. Jiang, J. He, J. Wu, X. Hu, Y. Ye, G. Christakos, “Assessing the severe eutrophication status and spatial trend in the coastal waters of Zhejiang province (China)”. Limnology and oceonography, vol. 64, No 1, pp. 3-17, agosto, 2018. https://doi.org/10.1002/lno.11013
- N.P. Kumudini, K. Sok, D.G. Avanthi, H. Yohey, C. Yoon, M. Raj, S. Binoy, S. Yong, “Fe(III) loaded chitosan-biochar composite fibers for the removal of phosphate from water”, Journal of Hazardous Materials, vol. 415, agosto, 2021. https://doi.org/10.1016/j.jhazmat.2021.125464.