Time series model for the characterization and prediction of the graduation rate at the University of Cartagena
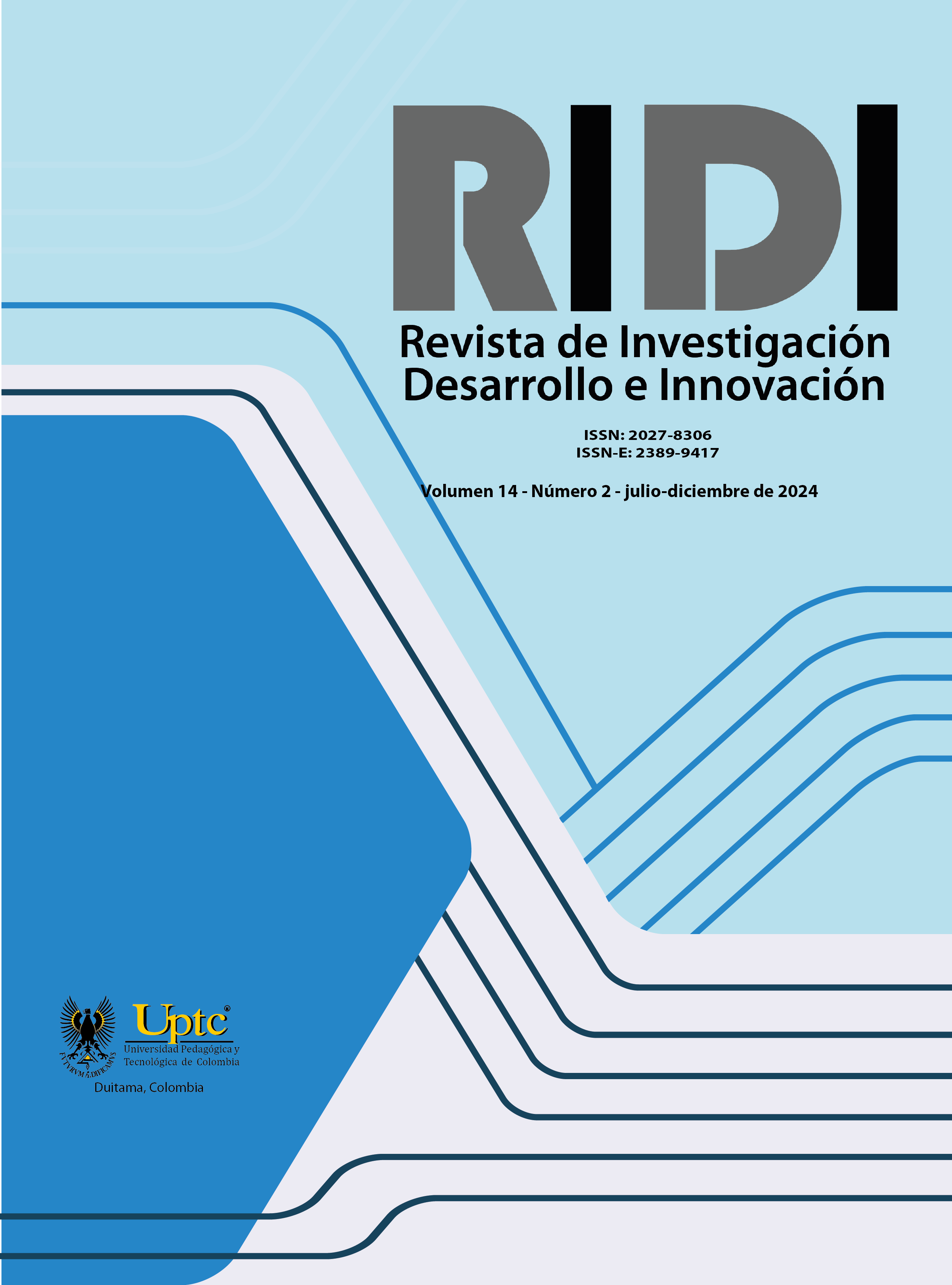
Abstract
For universities, characterizing graduation rates is a key indicator reflecting academic quality and the institution's ability to guide students towards successful entry into the workforce. This paper proposes a time series model for characterizing and predicting graduation rates at the University of Cartagena. Methodologically, the CRISP-DM methodology was adapted into four phases: P1. Business and data understanding, P2. Data preparation, P3. Model construction and evaluation, and P4. Deployment. As a result, various ARIMA models were implemented and evaluated to determine the best fitting model. This model serves as a reference for developing tools to support decision-making by university administrators regarding the number of graduating professionals, in line with educational quality standards.
Keywords
Graduates, graduation rate, predictive model, time series, ARIMA
Author Biography
Ana María Prieto-Romero
Ingeniera de Sistemas
Gabriel Elías Chanchí-Golondrino
Ingeniero en Electrónica y Telecomunicaciones , Doctor en Ingeniería Telemática.
Manuel Alejandro Ospina-Alarcón
Ingeniero de Control, Doctor en Ingeniería - Ciencia y Tecnología de Materiales.
References
- Bautista-Rodríguez, L. M., Jiménez, S. J., & Meza, J. J. (2015). Desempeño laboral de los enfermeros (a) egresados de la Universidad Francisco de Paula Santander. Revista Ciencia y Cuidado, 9(2), 64–70. https://doi.org/10.22463/17949831.340
- Bricio-Samaniego, K., Calle-Mejía, J., & Zambrano-Paladines, M. (2018). El marketing digital como herramienta en el desempeño laboral en el entorno ecuatoriano: estudio de caso de los egresados de la Universidad de Guayaquil. Universidad y Sociedad, 10 (4), 103–109.
- Calvillo, E. A., Mendoza, R., Munoz, J., Martinez, J. C., Vargas, M., & Rodriguez, L. C. (2016). Automatic algorithm to classify and locate research papers using natural language. IEEE Latin America Transactions, 14 (3), 1367–1371. https://doi.org/10.1109/TLA.2016.7459622
- Campos-Mesa, M. del C. (2008). Los egresados y su inserción laboral ¿estudias o trabajas? Revista Fuentes, 8, 322–332. http://hdl.handle.net/11441/54129
- Chan, R. Y.-Y., Wong, C. M. V., & Yum, Y. N. (2023). Predicting Behavior Change in Students With Special Education Needs Using Multimodal Learning Analytics. IEEE Access, 11, 63238–63251. https://doi.org/10.1109/ACCESS.2023.3288695
- Choe, N. H., & Borrego, M. (2019). Prediction of Engineering Identity in Engineering Graduate Students. IEEE Transactions on Education, 62 (3), 181–187. https://doi.org/10.1109/TE.2019.2901777
- Espinosa-Zúñiga, J. J. (2020). Aplicación de metodología CRISP-DM para segmentación geográfica de una base de datos pública. Ingeniería, Investigación y Tecnología, 21 (1), 1–13. https://doi.org/10.22201/fi.25940732e.2020.21n1.008
- Galgotia, D., & Lakshmi, N. (2021). Implementation of Knowledge Management with Artificial Intelligence in Higher Education. 2021 IEEE 6th International Conference on Computing, Communication and Automation (ICCCA), 832–836. https://doi.org/10.1109/ICCCA52192.2021.9666300
- García-Ancira, C., Treviño-Cubero, A., & Banda-Muñoz, F. (2019). Caracterización del seguimiento de egresados universitarios. Revista Estudios Del Desarrollo Social: Cuba y América Latina, 17 (1), 23–38. http://scielo.sld.cu/scielo.php?pid=S2308-01322019000100023&script=sci_arttext
- Jaramillo, A., Giraldo-Pinedo, A., & Ortiz-Correa, J. S. (2006). Estudio sobre egresados - La experiencia de la Universidad EAFIT. Revista Universidad EAFIT, 42 (141), 111–124. https://publicaciones.eafit.edu.co/index.php/revista-universidad-eafit/article/view/818/728
- Jie, L., Zheng, S., Qi, W., & Xiya, C. (2021). Analysis of Employment Status and Countermeasures of Biology Graduates in Local Normal Universities Based on Big Data Technology—Take the Graduates of Guangxi Normal University From 20l6 to 2020 as an Example. 2021 2nd International Conference on Artificial Intelligence and Education (ICAIE), 572–578. https://doi.org/10.1109/ICAIE53562.2021.00127
- Kashirina, I., Bondarenko, Y., & Azarnova, T. (2021). Analysis and Forecasting of the Market of Educational Services of the Region. 2021 1st International Conference on Technology Enhanced Learning in Higher Education (TELE), 30–34. https://doi.org/10.1109/TELE52840.2021.9482804
- Martinez-Plumed, F., Contreras-Ochando, L., Ferri, C., Hernandez-Orallo, J., Kull, M., Lachiche, N., Ramirez-Quintana, M. J., & Flach, P. (2021). CRISP-DM Twenty Years Later: From Data Mining Processes to Data Science Trajectories. IEEE Transactions on Knowledge and Data Engineering, 33 (8), 3048–3061. https://doi.org/10.1109/TKDE.2019.2962680
- Meng, S., Cai, J., Zhang, Y., Wei, J., Zheng, X., & Wang, J. (2022). Predictive Analysis of Higher Education Enrollment Rate in Cambodia Based on ARIMA Model. 2022 IEEE 2nd International Conference on Power, Electronics and Computer Applications (ICPECA), 220–223. https://doi.org/10.1109/ICPECA53709.2022.9719236
- Nudin, S. R., Warsito, B., & Wibowo, A. (2022). Impact of Soft Skills Competencies to predict Graduates getting Jobs Using Random Forest Algorithm. 2022 1st International Conference on Information System & Information Technology (ICISIT), 49–54. https://doi.org/10.1109/ICISIT54091.2022.9872669
- Padhan, P. C., & others. (2012). Application of ARIMA model for forecasting agricultural productivity in India. Journal of Agriculture and Social Sciences, 8 (2), 50–56.
- Schlesinger, W., Cervera, A., & Calderón, H. (2014). El papel de la confianza, la imagen y los valores compartidos en la creación de valor y lealtad: aplicación a la relación egresado-universidad. Revista Española de Investigación de Marketing ESIC, 18 (2), 126–139. https://doi.org/10.1016/j.reimke.2014.06.001
- Senay, S. (2015). On the impacts of project based learning for workplace preparedness of engineering graduates. 2015 10th System of Systems Engineering Conference (SoSE), 364–367. https://doi.org/10.1109/SYSOSE.2015.7151921
- Shekhar, P., Khan, T. H., Gajjar, S., & Duff, H. (2023). Investigating the predictive relationship of GPA on engineering students’ enrollment in entrepreneurial education programs: A Decision Tree Analysis. 2023 IEEE Frontiers in Education Conference (FIE), 1–4. https://doi.org/10.1109/FIE58773.2023.10342925
- Tahiru, F., & Parbanath, S. (2023). Using an Exploratory Analytical Approach to Distinguish the Habits of Graduating and Non-Graduating Students in a Virtual Learning Environment. 2023 International Conference on Artificial Intelligence, Big Data, Computing and Data Communication Systems (IcABCD), 1–8. https://doi.org/10.1109/icABCD59051.2023.10220542
- Uskov, V. L., Bakken, J. P., Byerly, A., & Shah, A. (2019). Machine Learning-based Predictive Analytics of Student Academic Performance in STEM Education. 2019 IEEE Global Engineering Education Conference (EDUCON), 1370–1376. https://doi.org/10.1109/EDUCON.2019.8725237
- Yong, S., Wang, Y., & Zhang, H. (2021). Higher Education Evaluation System Based on NET. 2021 6th International Conference on Smart Grid and Electrical Automation (ICSGEA), 307–310. https://doi.org/10.1109/ICSGEA53208.2021.00075