Application of New Fuzzy Measure in Multi-Attribute Decision-Making
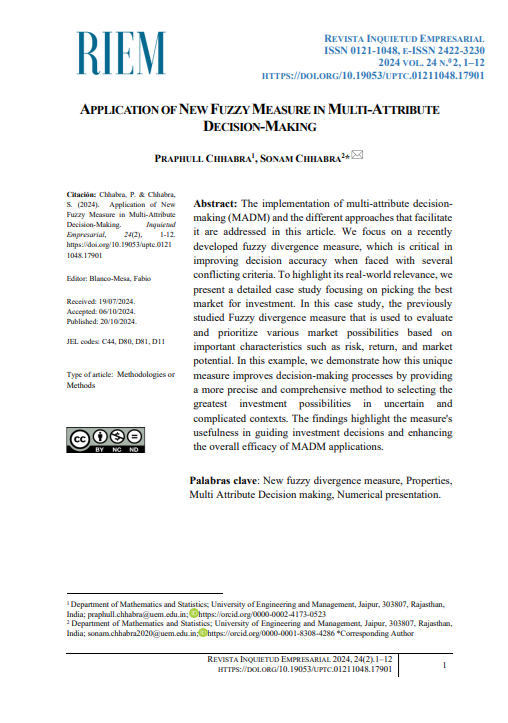
Abstract
The implementation of multi-attribute decision-making (MADM) and the different approaches that facilitate it are addressed in this article. We focus on a recently developed fuzzy divergence measure, which is critical in improving decision accuracy when faced with several conflicting criteria. To highlight its real-world relevance, we present a detailed case study focusing on picking the best market for investment. In this case study, the previously studied Fuzzy divergence measure that is used to evaluate and prioritize various market possibilities based on important characteristics such as risk, return, and market potential. In this example, we demonstrate how this unique measure improves decision-making processes by providing a more precise and comprehensive method to selecting the greatest investment possibilities in uncertain and complicated contexts. The findings highlight the measure's usefulness in guiding investment decisions and enhancing the overall efficacy of MADM applications.
JEL Codes: C44, D80, D81, D11
Received: 19/07/2024. Accepted: 06/10/2024. Published: 20/10/2024.
Keywords
New fuzzy divergence measure, Properties, Multi Attribute Decision Making, Numerical presentation
References
- Bajaj, R. K., & Hooda, D. S. (2010). On some new generalized measures of fuzzy information. World Academy of Science, Engineering and Technology, 38, 747–752.
- Bozorg‐Haddad, O., Loáiciga, H., & Zolghadr‐Asli, B. (2021).
- Handbook on Multi‐Attribute Decision‐Making Methods. https://doi.org/10.1002/9781119563501 DOI: https://doi.org/10.1002/9781119563501
- Bhandari, D., & Pal, N. R. (1993). Some new information measures for fuzzy sets. Information Sciences, 67(3), 209–228. https://doi.org/10.1016/0020-0255(93)90073-u DOI: https://doi.org/10.1016/0020-0255(93)90073-U
- Celik, E., Gul, M., Yucesan, M., & Mete, S. (2019). Stochastic multi-criteria decision-making: an overview to methods and applications. Beni-Suef University Journal of Basic and Applied Sciences, 8(1). https://doi.org/10.1186/s43088-019-0005-0. DOI: https://doi.org/10.1186/s43088-019-0005-0
- Chhabra, P., & Chhabra, S. (2023). A new fuzzy divergence measure and its properties. In Proceedings of the 1st International Conference on Computational Applied Sciences and Its Applications (AIP Conf. Proc. 2768, pp. 020030-1–020030-8). ICCASA, Jaipur, India. https://doi.org/10.1063/5.0148323 DOI: https://doi.org/10.1063/5.0148323
- Criado, F., & Gachechiladze, T. (1997). Entropy of fuzzy events. Fuzzy Sets and Systems, 88(1), 99–106. https://doi.org/10.1016/s0165-0114(96)00073-5 DOI: https://doi.org/10.1016/S0165-0114(96)00073-5
- De Luca, A., & Termini, S. (1972). A definition of a nonprobabilistic entropy in the setting of fuzzy sets theory. Information and Control, 20(4), 301–312. https://doi.org/10.1016/s0019-9958(72)90199-4 DOI: https://doi.org/10.1016/S0019-9958(72)90199-4
- Jain, K. C., & Chhabra, P. (2014). Bounds of new f-divergence measure by using new information inequality. Antarctica Journal of Mathematics, 11(1), 55–68.
- Kahraman, C. (2008). Multi-criteria decision-making methods and fuzzy sets. In Springer Optimization and Its Applications (pp. 1–18). https://doi.org/10.1007/978-0-387-76813-7_1 DOI: https://doi.org/10.1007/978-0-387-76813-7_1
- Montes, S., Couso, I., Gil, P., & Bertoluzza, C. (2002). Divergence measure between fuzzy sets. International Journal of Approximate Reasoning, 30(2), 91–105. https://doi.org/10.1016/s0888-613x(02)00063-4 DOI: https://doi.org/10.1016/S0888-613X(02)00063-4
- Ohlan, A. (2015). A New Generalized Fuzzy Divergence Measure and Applications. Fuzzy Information and Engineering, 7(4), 507–523. https://doi.org/10.1016/j.fiae.2015.11.007 DOI: https://doi.org/10.1016/j.fiae.2015.11.007
- Pal, N., & Bezdek, J. (1994). Measuring fuzzy uncertainty. IEEE Transactions on Fuzzy Systems, 2(2), 107–118. https://doi.org/10.1109/91.277960 DOI: https://doi.org/10.1109/91.277960
- Pal, N. R., & Pal, S. K. (1989). Object-background segmentation using new definitions of entropy. IEE Proceedings E (Computers and Digital Techniques), 136(4), 284–295. https://doi.org/10.1049/ip-e.1989.0039 DOI: https://doi.org/10.1049/ip-e.1989.0039
- Parkash, O., & Gandhi, C. P. (2010). Applications of trigonometric measures of fuzzy entropy to geometry. World Academy of Science, Engineering and Technology, 37, 468–471.
- Rani, P., Govindan, K., Mishra, A. R., Mardani, A., Alrasheedi, M., & Hooda, D. S. (2020b). Unified Fuzzy Divergence Measures with Multi-Criteria Decision Making Problems for Sustainable Planning of an E-Waste Recycling Job Selection. Symmetry, 12(1), 90. https://doi.org/10.3390/sym12010090 DOI: https://doi.org/10.3390/sym12010090
- Rényi,A.(1961).On measures of entropy and information. In Proceedings of the Fourth Berkeley Symposium on Mathematics, Statistics, and Probability, Vol. I (pp. 547–562). University of California Press.
- Shannon, C. E. (1948). A Mathematical Theory of Communication. Bell System Technical Journal, 27(3), 379–423. DOI: https://doi.org/10.1002/j.1538-7305.1948.tb01338.x
- Umar, A., & Saraswat, R. N. (2021). New Generalized Intuitionistic Fuzzy Divergence Measure with Applications to Multi-Attribute Decision Making and Pattern Recognition. Recent Advances in Computer Science and Communications, 14(7), 2247–2266. https://doi.org/10.2174/2666255813666200224093221 DOI: https://doi.org/10.2174/2666255813666200224093221
- Verma ,R.,& Sharma, B.D. (2011). On generalized exponential fuzzy entropy. World Academy of Science, Engineering and Technology, International Journal of Mathematical, Computational, Physical, Electrical and Computer Engineering, 5(12), 1895–1898.
- Verma, R., & Sharma, B. D. (2014). A New Inaccuracy Measure for Fuzzy Sets and its Application in Multi-Criteria Decision Making. International Journal of Intelligent Systems and Applications, 6(5), 62–69. https://doi.org/10.5815/ijisa.2014.05.06 DOI: https://doi.org/10.5815/ijisa.2014.05.06
- Verma, R., & Dev, B. (2015). Hesitant fuzzy geometric Heronian mean operators and their application to multi-criteria decision making. Scientiae Mathematicae Japonicae, 281, 23–39.
- Yager, R. R. (2001). A general approach to uncertainty representation using fuzzy measures. In The Florida AI Research Society (pp. 619–623).
- Zadeh, L. (1968). Probability measures of Fuzzy events. Journal of Mathematical Analysis and Applications, 23(2), 421–427. DOI: https://doi.org/10.1016/0022-247X(68)90078-4
- Zadeh, L. A. (2002). Toward a generalized theory of uncertainty (GTU): An outline. Information Sciences: Informatics and Computer Sciences, 172(1), 1–40. DOI: https://doi.org/10.1016/j.ins.2005.01.017
- Zadeh, L. (1965). Fuzzy sets. Information and Control, 8(3), 338–353. https://doi.org/10.1016/s0019-9958(65)90241-x DOI: https://doi.org/10.1016/S0019-9958(65)90241-X